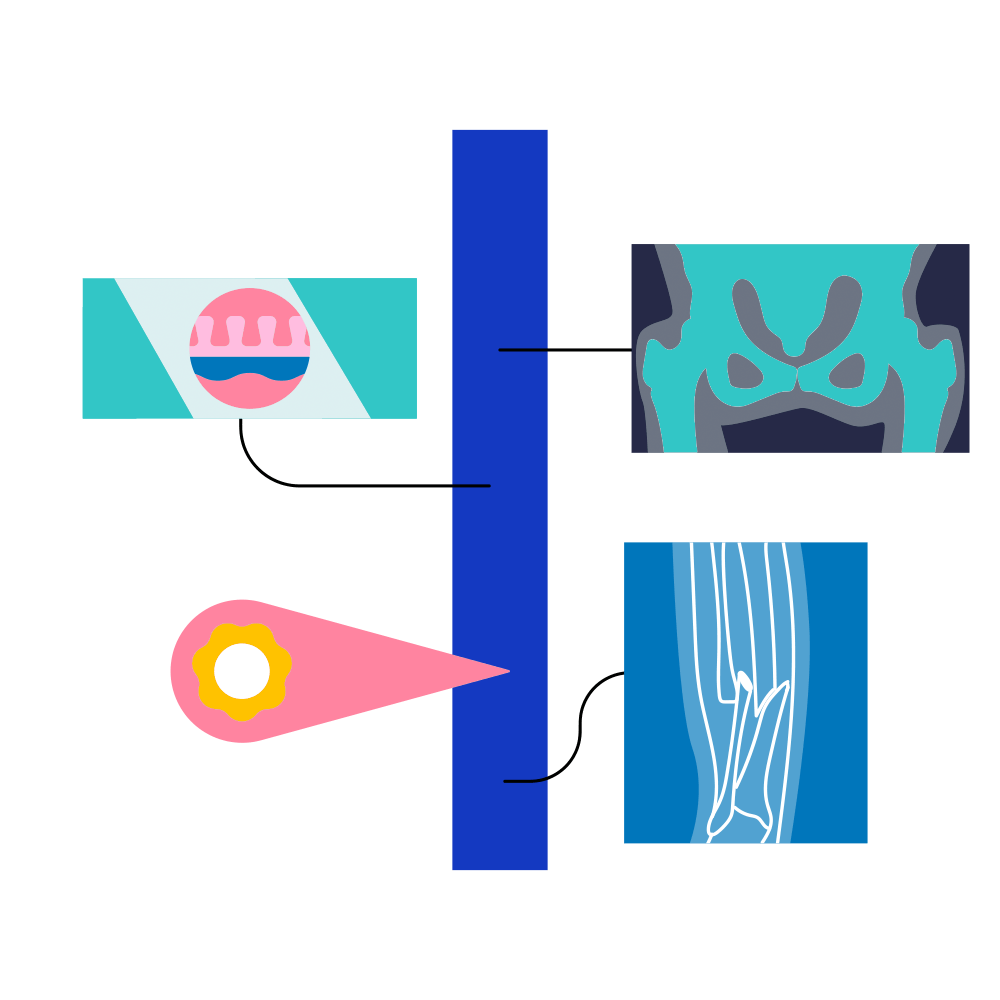
Imaging Data
Imaging data refers to the ‘results’ (i.e., the images or pictures) produced by medical imaging tests such as ultrasound, CT scans, MRI scans, PET scans and X-rays. Most people are familiar with the image of an unborn baby produced by an ultrasound scan when a person is pregnant. In this example, the ultrasound is the test and the picture generated from the scan of the baby is the image data.
Here’s how this data is used for machine learning:
- Researchers gather many images of different people (for example, CT scans) so they can be used to explore specific medical questions.
- First, a human looks at all the images, and labels different sections of each image as to whether they contain a feature of interest (e.g., contains a tumour or not).
- Then these labelled images are centralised and organised in a single ‘dataset’ so they can be used in machine learning.
- This dataset is then used to train diagnostic algorithms to automatically detect potential medical issues (e.g. accurately spotting the presence of a tumour) by analysing image data.
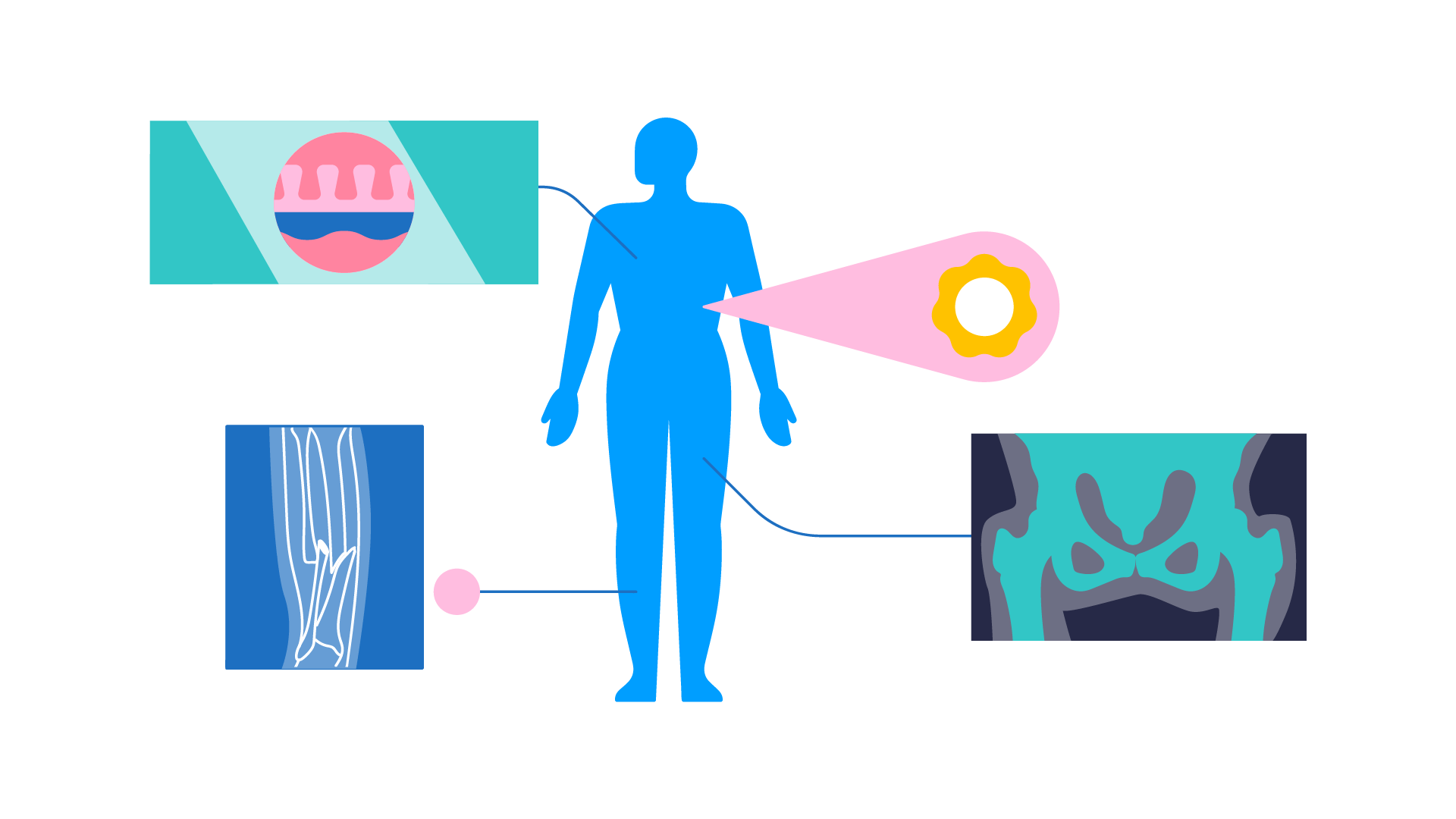
A simplified version of this process is familiar to many people because of ‘CAPTCHA’ tests (or ‘are you a robot’ tests) that ask people to select all parts of an image that show a specific thing (e.g., a car). Algorithms that are shown hundreds of correctly labelled images can then learn how to accurately identify everyday objects, like cars.
In a medical setting, algorithms can learn how to accurately identify normal and abnormal scans in real-time far faster than a human could. These algorithms are transformed into practical AI tools that can be used by radiographers (clinicians who read scans in hospital settings) to accelerate the process of diagnosing patients, playing a crucial role in facilitating earlier intervention which may lead to better patient outcomes.
Imaging data refers to the results (i.e., the images) produced by imaging tests such as computed tomography scans (CT scans), magnetic resonance imaging scans (MRI scans) magnetic resonance angiography scans (MRA scans), mammography scans, nuclear scans, positron emission tomography (PET) scans, X-Rays, and ultrasounds. The individual images can be aggregated, curated (quality assured, anonymised, segmented, de-noised), harmonised and annotated to create large databases for the training and validation of image-based diagnostic algorithms.
Annotation is the most crucial stage of this preparation process, involving the labelling of individual elements in the images to highlight what ‘normal’ and ‘abnormal’ looks like, as well as what is useful or ignorable information. Algorithms trained on hundreds of these annotated images, contained within large databases as described above, can learn to identify abnormalities (such as tumours) as well – if not better than – human radiographers.
This diagnostic use case of AI has already been proven to be highly successful. There are more than 400 regulatory approved imaging algorithms in use in the United States and many more available on the market elsewhere. These algorithms have shown to be capable of impressive accuracy and sensitivity not just to the identification of abnormalities but also to subtle changes in tumours which can give early indication as to whether a particular treatment protocol is working as expected.
There are several reasons why imaging diagnostic algorithms have had more success so far than diagnostic algorithms trained on other types of data such as electronic health record (EHR) data. However, the primary reason is greater data standardisation. Whilst EHR data is often collected and stored in multiple different formats that are not necessarily comparable, most imaging data conform to the internationally standardised DICOM (Digital Imaging and Communications in Medicine) format which ensures interoperability (or easy comparability) between data produced in different settings. The wide adoption of this standard has made it considerably easier to create ‘ground truth’ (or gold standard) imaging datasets than it has been to create ground truth EHR datasets.
An Owkin example
PULS-AI – a research project led by Owkin – trained a machine learning model on CT scans, ultrasound images, and clinical data from more than 600 patients from 17 French treatment centres, to predict the outcome of patients with seven different cancers treated with antiangiogenics. The results of the study, published in the European Journal of Cancer, showed that the model was able to accurately categorise patients into different groups with different likely prognostic outcomes.
Further reading
- Kondylakis, Haridimos et al. 2023. ‘Data Infrastructures for AI in Medical Imaging: A Report on the Experiences of Five EU Projects’. European Radiology Experimental 7(1): 20.
- Oren, Ohad, Bernard J Gersh, and Deepak L Bhatt. 2020. ‘Artificial Intelligence in Medical Imaging: Switching from Radiographic Pathological Data to Clinically Meaningful Endpoints’. The Lancet Digital Health 2(9): e486–88.