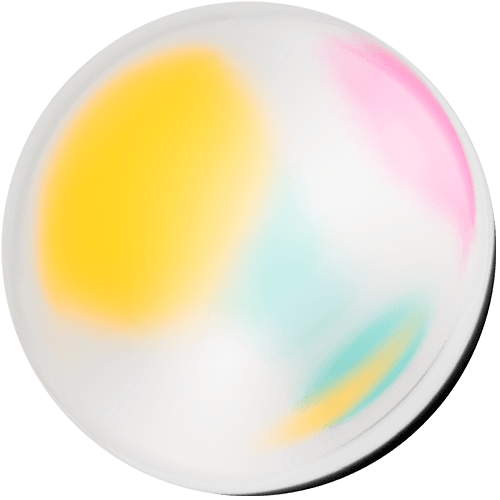
AI drug positioning engine
Applying AI to match a drug asset with new indications or subpopulation of existing indications
Challenge
90% of blockbuster medicines only work for 30-50% of patients
- Lack understanding of TME and drug mechanism of action
- Incomplete knowledge of clinical heterogeneity
- Testing all possible drug combinations is impractical
Solution
Owkin’s DrugMATCH
- DrugMATCH is a suite of end-to-end tools that uses knowledge graphs, graph machine learning, biomedical literature and multimodal patient data to identify novel disease indications and associated subgroups for a given drug
AI Drug Positioning for New Patient Benefits
[
02:59
]
Discover how Owkin's AI-driven Drug Match Engine finds new uses for existing drugs by analyzing knowledge graphs and patient data. Learn about its innovative machine-learning techniques to predict new indications, patient subgroups, and enhance treatment efficacy.
Drug positioning engine
How does DrugMATCH work?
DrugMATCH can be used for AI drug repurposing, also know as drug positioning, or indication or label expansion, which involves using AI to find new therapeutic uses for existing drugs. We apply DrugMATCH to drug positioning in immunology, oncology and other therapy areas.
Best-in-class data
Broad data access
We access patient data for hundreds of disease indications from public datasets.
We integrate this data with biomedical literature and multimodal patient data from our network of partners

Knowledge graph
Drug asset in broad disease space
Our knowledge graph represents known interactions between drugs, targets and indications.
- Tens of thousands of nodes
- Millions of connections between nodes

AI drug-disease matching
Prioritization of best drug-disease pairs
Our graph ML methods learn the patterns that made drugs/targets effective during past clinical trials.
We use AI to predict the best disease indications to potentially target with the drug of interest.
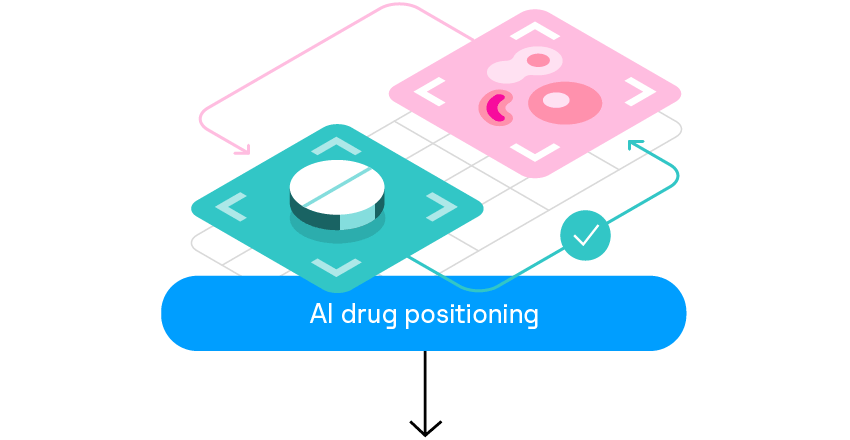
AI subgroup matching
Positioning of drug on patient subpopulations
Deep dive into a given drug-disease pair, to characterize the patient subtypes most likely to respond to that drug.
- Owkin data access
- Multimodal patient embeddings
- ML subpopulation optimization
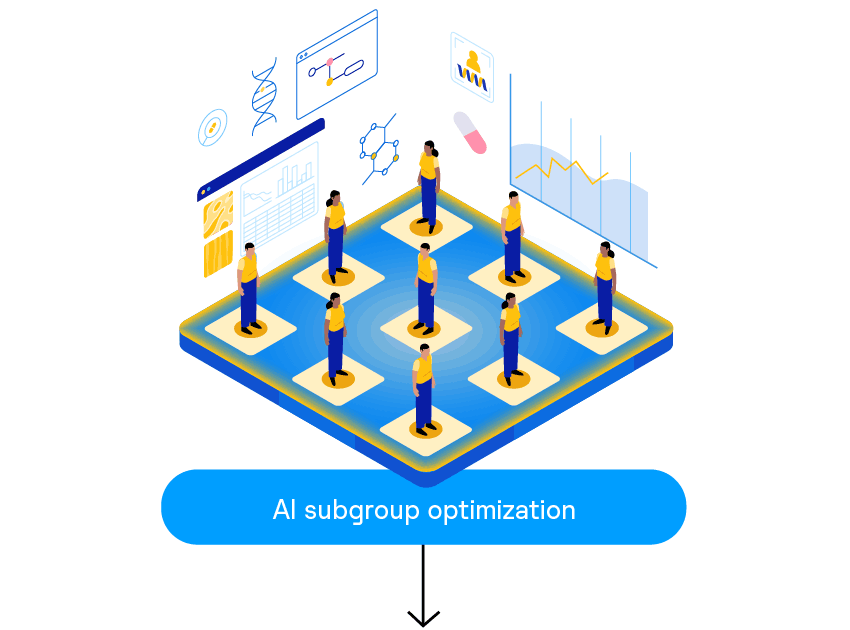
Discovery
- Top indications potentially affected by the drug
- Matched patient subpopulations to increase treatment efficacy

Subgroup discovery
Multimodal AI-powered biomarkers
We combine cutting-edge machine learning and biology to identify biomarkers.
TargetMATCH
A new approach to target discovery
A suite of end-to-end tools that uses multimodal patient data as the input, and outputs the top candidate targets and paired patient subgroups that would most benefit from therapeutic intervention on these targets.