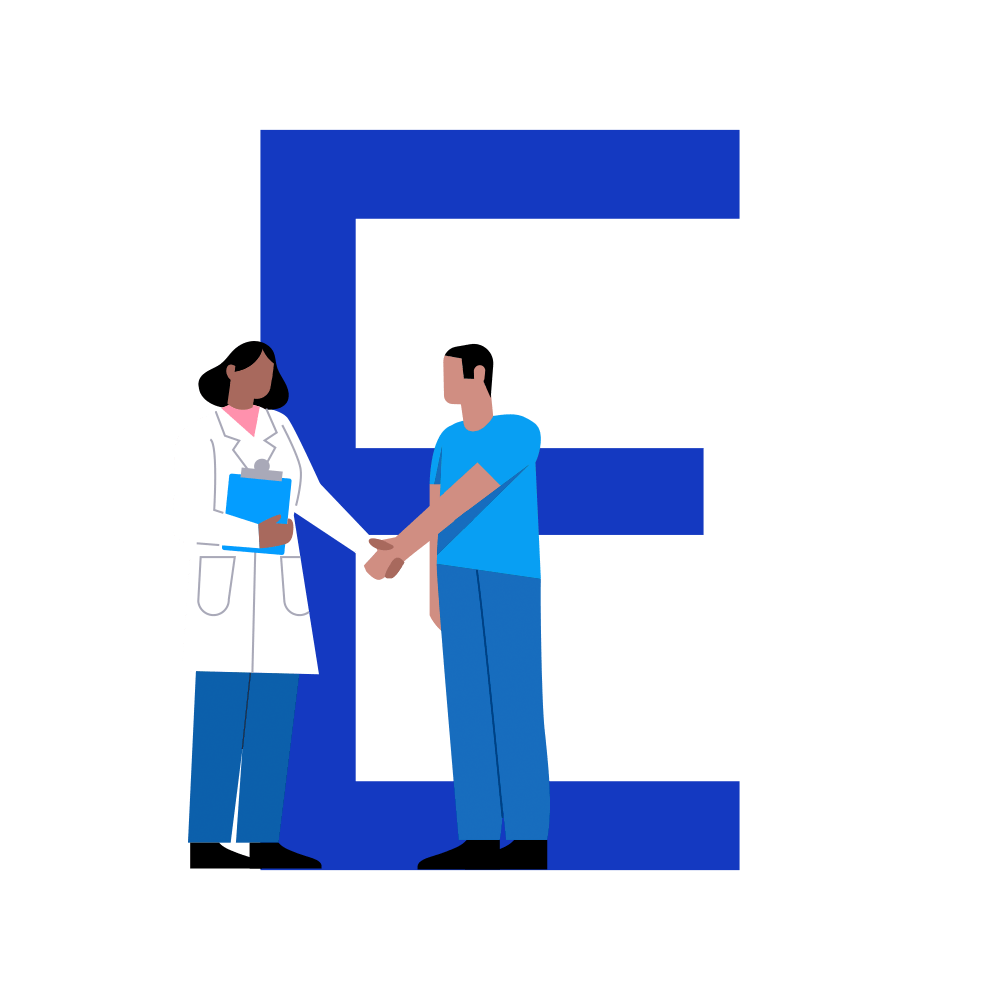
Ethics
Most people are familiar with the oath doctors take to ‘Do No Harm’ to the people that they look after. This is the founding principle of medical ethics: the moral guidelines that help doctors and medical staff make decisions about what they should and should not do when looking after someone’s health.
The core guidelines are:
- Beneficence: act with the best intentions of the patient
- Non-maleficence: protect the patient from harm
- Autonomy: help the patient understand what is happening to them and ensure they are involved in all decisions about their care
- Justice: ensure all patients of equal need are treated in the same way
In physical medicine, there are lots of rules that help doctors meet these ethical requirements. For example, when a patient is having an operation, they have to give informed consent (i.e., they are told what will happen to them, what the risks and benefits are), and the operation should be scheduled according to priority (i.e., the patients with the greatest need should get to the operating room first). This practice upholds all four medical ethics principles.
In the context of AI, it can sometimes be harder to interpret the principles. What does it mean, for example, to cause no harm to a person if nothing is physically happening to their body? This does not mean interpreting the ‘Do No Harm’ principles is impossible though, and abiding by ethical principles is still very important for all those involved in developing and using AI for healthcare.
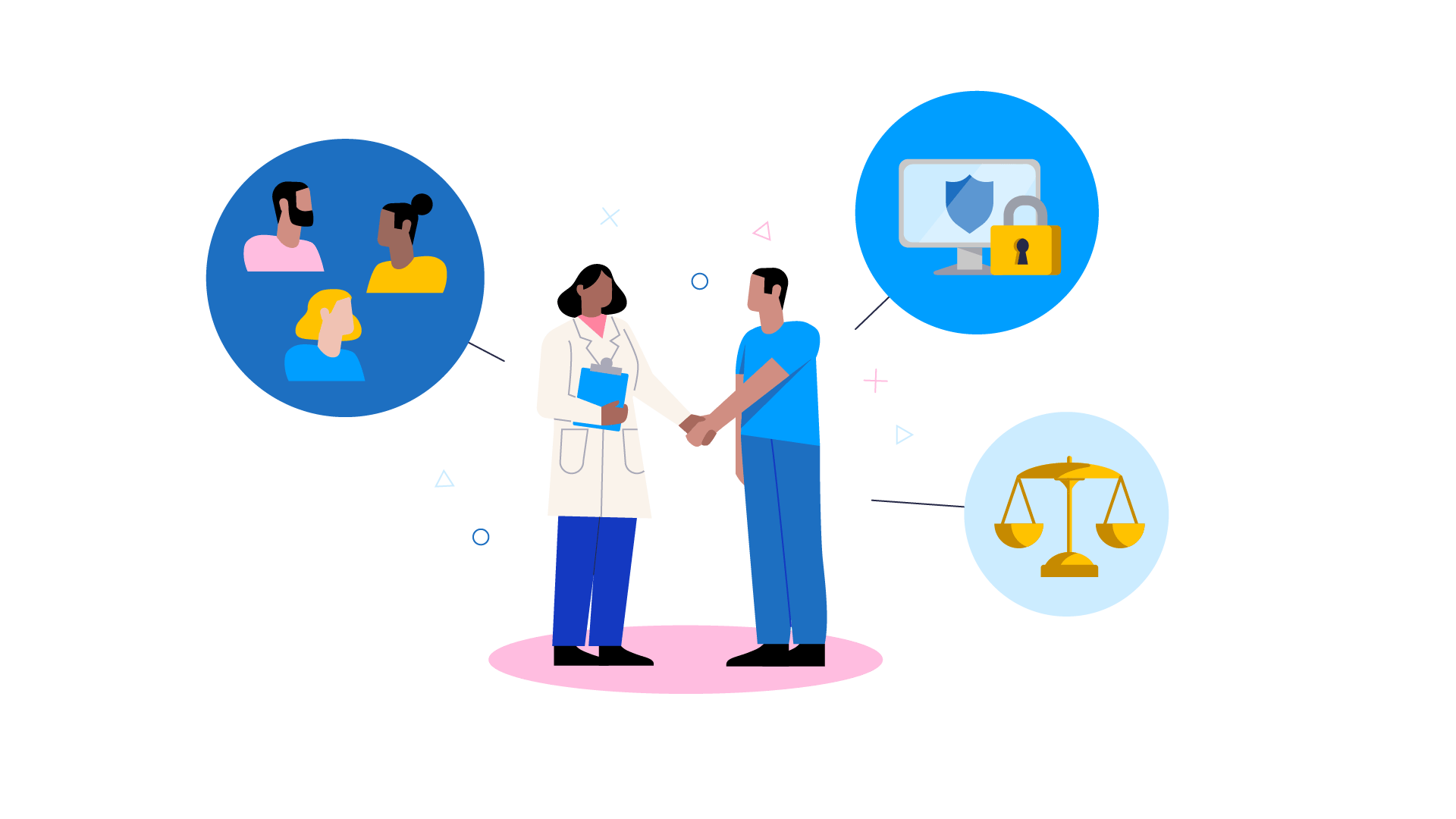
Here are some examples of what this might look like:
Beneficence
To act with a person’s best interests at heart, means that all aspects of a person’s life should be considered when a medical decision is made. Just because a drug has proved to be the most effective at treating a particular type of cancer, does not mean it is the best drug for that patient if the side effects are incompatible with that person’s life. If an algorithm is used to advise doctors on how to treat a patient, it is important that a wide range of factors such as what their priorities are, where they live, and what their home life is like are taken into consideration as well.
Non-maleficence
To protect patients from harm in the context of AI, means to protect them from the harms of privacy infringement, overdiagnosis, or psychological harm.
- Privacy
Medical information (such as what conditions a person has been diagnosed with) is very sensitive. If it is leaked to the wrong person or organisation, significant harm could come to that patient. For example, they could be denied insurance or they could experience discrimination. It is critical that their privacy is protected at all times when any form of patient data is being used in the context of AI. - Overdiagnosis
The process of diagnosing a patient can sometimes be tiring and painful. Patients should only be subject to tests (e.g. blood tests or biopsies), when it is absolutely necessary and it’s known that a diagnosis will lead to better outcomes. This means that algorithms should not be used to ‘screen’ patients for abnormalities or conditions that may cause no harm if left untreated as the harm from the process of diagnosis might be worse. - Psychological harm
A person can also experience psychological harm if, for instance, they are informed that they might one day develop a life-threatening disease and this causes them to worry or to feel excessively anxious. For example, when algorithms are being used to predict a patient’s risk of developing diseases, the impact on that person’s psychological well being (or their mental health) should be factored in. If a person is predicted to be at high risk of developing a particular disease and nothing can be done to reduce that risk, it might be best to not tell the patient and instead advise them simply to come for regular testing.
Autonomy
To ensure patients are able to exert control over their own healthcare, and to know what is happening to them, there should be transparency around who has had access to their data for what purpose. This enables patients to give informed consent to any form of AI-powered diagnosis or treatment recommendation and to be able to ‘understand’ how an algorithm has reached a decision about their care.
Justice
If the provision of healthcare is to be just, it needs to be fair. In the context of AI, this means it is important to be aware of data bias. If an algorithm is trained on biased data, it will likely be ‘unfair’ because it may be more accurate for some patients than for others. This could cause problems if, for instance, it meant that one group of patients were regularly being misdiagnosed.
Medicine, despite being one of the most highly regulated fields in existence, is not purely governed by rules, regulations, policies, and standards. It also has a long history of ethical governance from the Hippocratic Oath to ‘do no harm’, to the introduction of bioethics principles to, more recently, medicine-adjacent ethical interventions such the Bermuda Principles, intended to govern human genome sequencing.
It’s necessary to ensure the introduction of AI is also subject to rigorous ethical analysis, alongside technical, regulatory, and sociocultural analysis. This can be done by, firstly, applying the expanded list of bioethics principles (autonomy, beneficence, non-maleficence, justice, explainability to the analysis of the ethics of AI for healthcare, and, secondly, by considering the broader value-based implications:
‘Autonomy’ broadly refers to the ability of a person to make their own life. It is a key concept in Western moral and political philosophy and is protected/harmed by a person’s ability/inability to self-govern in a manner that is free from external control and undue interference.
To a large extent, autonomy is now seen as the ‘primary principle’ and the need to protect autonomy has been attached to several significant shifts in modern medicine, such as the movement from ‘paternalistic care’ towards ‘patient-centred care’.
AI relies on large volumes of data, including data that patients may collect themselves (e.g.,from smartwatches or shopping records) and it has the ability to predict risk with the intention of encouraging preventative action. This means that, without careful thought, AI has significant potential to nudge and police individuals into behaving in ways that do not necessarily align with their own personal values in the name of pursuing ‘optimum health.’ The fact that much of this algorithmic nudging happens within a black box, and involves the evaluation of an individual against often inscrutable baselines, amplifies the potential for AI to have a negative impact on autonomy.
Although recent AI technology can potentially be empowering for individuals (such as learning from smartwatch use), a future age of near continuous unobservable screening processes may impinge on their right to informed and meaningful consent along with their right to ‘not know’ if they think certain health information (such as that involving future risk) might cause them psychological harm.
‘Beneficence’ broadly refers to the duty of healthcare providers to both prevent/remove harm and to promote wellbeing/welfare. This involves more than ‘just’ identifying a diagnosis that fits a list of quantifiable symptoms and matching this to an ‘effective’ drug, or identifying potential risk factors. Beneficent care also involves seeing the person as a whole (taking into account their personal beliefs, values, etc.), shared decision-making, and providing care in an empathetic, compassionate, and trustworthy manner.
Whilst AI might be able to mimic human empathy, it cannot truly ‘understand’ it and therefore might not be able to completely replicate its effects. Furthermore, there is a growing concern that AI’s reliance on ‘quantifiable’ data might lead to the exclusion of other ‘data’ about a patient’s life in the decision-making process. For these reasons, it is important to see AI as a helpful aid, but not a replacement for clinicians who are capable of contextualising ‘evidence’ and focusing on the more ‘holistic’ aspects of care.
‘Non-maleficence’ is the principle most closely linked to the Hippocratic ‘Do No Harm’ oath. Here, the concerns raised by AI mostly stem from its ability to do more harm than good. It could potentially infringe on patient privacy, lead to overdiagnosis that can cause both physical and psychological harm as well as result in waste, or enable healthcare to be unethically manipulated by economic and market forces.
‘Justice’ is the most familiar of the ethical principles in the context of AI, at least in the public domain, given its close tie with issues of bias. The ethical concerns here are that issues with the way medical data used to train AI are collected, curated, and interpreted may lead to biased algorithms which, over time, might lead to discrimination. Whilst most of the focus in the literature and in the press in this domain has been on the potential for AI to be biased in terms of sex, gender, or race, there are also lesser-known bias problems.
Precision medicine (enabled by AI) has the potential to divide the population into ‘good patients’ deserving of care (those who respond well to treatments and act on preventive advice) and ‘bad patients’ undeserving of care (those who do not respond well to treatments and may be unable to act on preventive advice). This could lead to latent bias (i.e., bias that develops over time) and the potential for AI to amplify the effects of the inverse care law (i.e., those who are in greatest need of care are least able to access it).
If the widespread implementation of AI is to be ‘successful’, then it will be necessary to question any assumptions that algorithms are somehow more objective than humans, and to develop a range of mechanisms for dealing with the sources of bias and identifying the consequences.
An Owkin example
In this essay, Jessica Morley, Director of Policy for the Bennett Institute for Applied Data Science, discusses the unique ethical challenges of applying AI in medical contexts:
“Healthcare is an amazing dichotomy. On the one hand, you’re dealing with a really, really physical thing—with human bodies, with emotions, with this incredibly personal part of life—but then, on the other hand, you’re dealing with abstraction, with data, with science.
At its heart, healthcare is one of the most human professions there is. Now, algorithms can absolutely empower diagnostics—they are supremely efficient at finding abnormalities. But they cannot have conversations, they cannot hold a hand, they cannot administer end-of-life care with compassion. In everything we do, we need to make sure these human bonds are not impacted. In fact, AI should be about freeing medical professionals up to focus on these human things.”
Further reading
- Avellan, Tero, Sumita Sharma, and Markku Turunen. 2020. ‘AI for All: Defining the What, Why, and How of Inclusive AI’. In Proceedings of the 23rd International Conference on Academic Mindtrek, AcademicMindtrek ’20, New York, NY, USA: Association for Computing Machinery, 142–44
- Chin-Yee, Benjamin, and Ross Upshur. 2019. ‘Three Problems with Big Data and Artificial Intelligence in Medicine’. Perspectives in Biology and Medicine 62(2): 237–56.
- Cirillo, Davide et al. 2020. ‘Sex and Gender Differences and Biases in Artificial Intelligence for Biomedicine and Healthcare’. npj Digital Medicine 3(1): 81.
- Grote, Thomas, and Philipp Berens. 2020. ‘On the Ethics of Algorithmic Decision-Making in Healthcare’. Journal of Medical Ethics 46(3): 205–11.
- Heyen, Nils B., and Sabine Salloch. 2021. ‘The Ethics of Machine Learning-Based Clinical Decision Support: An Analysis through the Lens of Professionalisation Theory’. BMC Medical Ethics 22(1): 112.
- Kerasidou, Angeliki. 2020. ‘Artificial Intelligence and the Ongoing Need for Empathy, Compassion and Trust in Healthcare’. Bulletin of the World Health Organization 98(4): 245–50.
- McCradden, Melissa D et al. 2020. ‘Patient Safety and Quality Improvement: Ethical Principles for a Regulatory Approach to Bias in Healthcare Machine Learning’. Journal of the American Medical Informatics Association 27(12): 2024–27.
- McDougall, Rosalind J. 2019. ‘Computer Knows Best? The Need for Value-Flexibility in Medical AI’. Journal of Medical Ethics 45(3): 156–60.
- Morley, Jessica, and Luciano Floridi. 2020. ‘The Limits of Empowerment: How to Reframe the Role of MHealth Tools in the Healthcare Ecosystem’. Science and Engineering Ethics 26(3): 1159–83.
- Morley, Jessica et al. 2020. ‘The Ethics of AI in Health Care: A Mapping Review’. Social Science & Medicine 260: 113172.
- Rubeis, Giovanni. 2023. ‘Liquid Health. Medicine in the Age of Surveillance Capitalism’. Social Science & Medicine 322: 115810.
- Schwartz, Peter H., and Eric M. Meslin. 2008. ‘The Ethics of Information: Absolute Risk Reduction and Patient Understanding of Screening’. Journal of General Internal Medicine 23(6): 867–70.