
Data-driven covariate adjustment
Optimizing randomized control arm clinical trials
Using AI-discovered prognostic covariates to strengthen treatment signal
Question
How do I ensure my trial meets its primary endpoint by increasing power without adding more patients?
By adjusting for data-driven covariates in the statistical analysis of your trial, you can reduce bias and increase the power of your study without having to increase sample size.
Owkin’s solution
Data-driven covariate adjustment
Owkin’s data-driven covariate adjustment increases the probability of phase III trial success through increased statistical power and broader inclusion criteria.
Methodology
Click
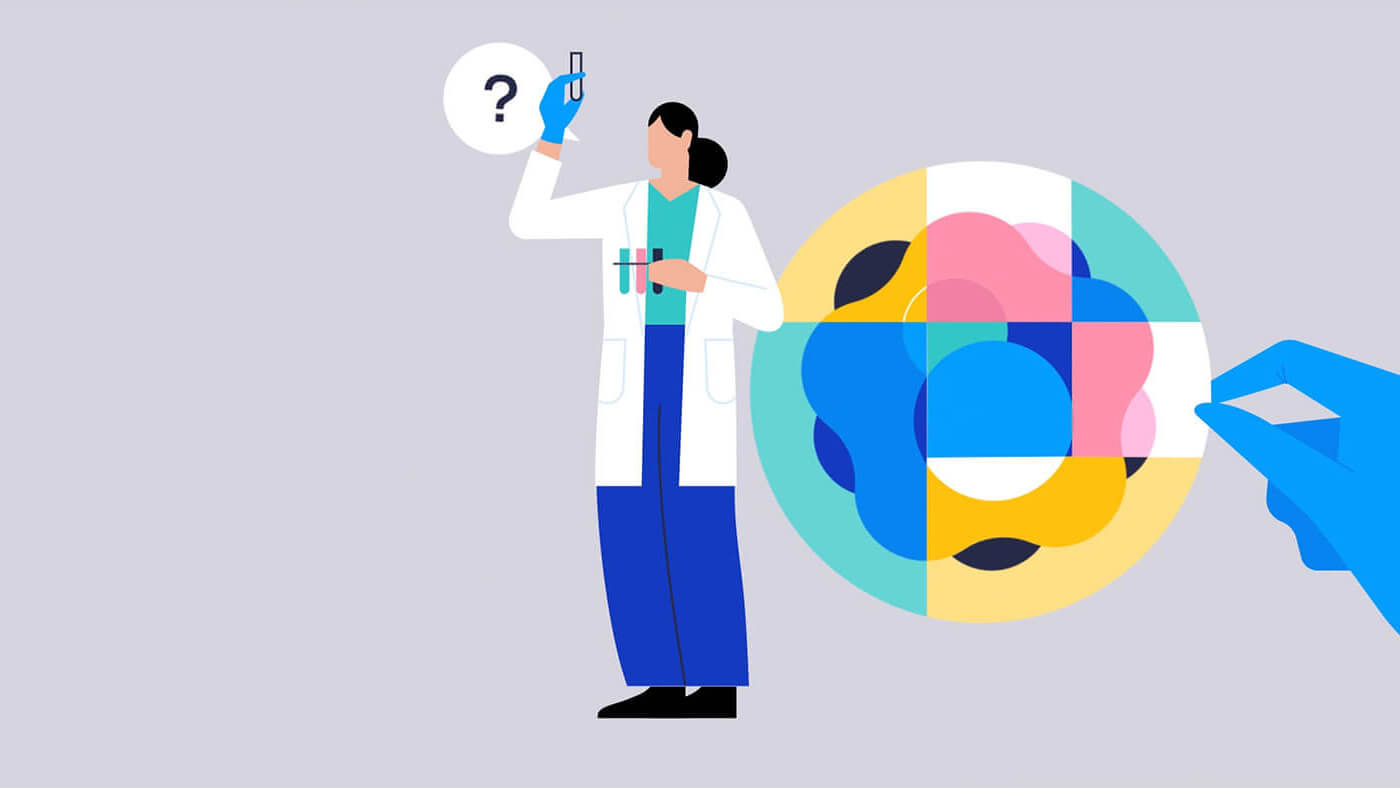
1
the target indication and inclusion criteria being studied
Selection of criteria to build external training data
Step 1
Understand
the target indication
Click

2
data hunt
Build the external training data set to accurately match the trial inclusion criteria
Step 2
Data hunt
to build external training data set
Click

3
Combine image and clinical features to train a model to identify which pre-treatment covariates best predict patient outcomes
Step 3
State-of-the-art ML
to select prognostic covariates
Click

4
Either:
1. Apply the selected prognostic covariates for adjustment
2. Directly use the model as a composite covariate during adjustment
1. Apply the selected prognostic covariates for adjustment
2. Directly use the model as a composite covariate during adjustment
Step 4
Covariate adjustment
to improve trial readouts
“A main potential strength of the artificial intelligence (AI) models is the gain in prognostic performance compared to an adjustment with covariates used in current practice in clinical trial settings. This gain in performance could translate to gains in statistical power.”
European Medicines Agency
Letter in support of Owkin's approach