MELLODDY: A 'co-opetitive' machine learning platform powered by Owkin
In healthcare, the cost needed to bring a new drug to market is staggering: €1.9 billion and 13 years on average! These figures alone, help explain the critical mass of pharmaceutical companies. But even for such large companies, economies of scale do not counterbalance the rising R&D costs and challenges in the pharmaceutical industry. Today more than ever, innovation and optimization are crucial to increase the pharmaceutical industry’s productivity and sustainability.
Artificial Intelligence and Machine Learning in general are poised to optimize nearly all the steps in the drug design pipeline. One concrete example of a promising approach to realize time and cost efficiencies is the partial virtualization of drug discovery by machine learning. Models are trained to predict the outcome of test compounds in wet-lab experiments; for example, evaluating how strongly a given compound binds to a given protein. Such models can then be used to inform the discovery process by suggesting more promising compounds to pursue.
As with any ML model application, predictive performance depends on the availability of enough qualitative training data. Importantly, for decades pharmaceutical companies have all invested tremendous efforts in collecting vast amounts of data points, significantly surpassing the quality and quantity of public datasets today. But for reasons of competitivity, companies only model their own data; until now.

In the framework of the Innovative Medicine Initiative, some of the world’s largest pharmaceutical companies jointly launched a call for a platform to leverage each other’s data for building predictive models for drug discovery, without comprising data privacy. These 10 pharmaceutical companies intend to collectively train predictive models on an unprecedented volume of data. The collective dataset will comprise more than 10 million annotated small molecules, more than 1 billion activity labels measured in biological assays, and multiple high-complexity phenotypes at high throughput. Put simply, the dataset size is likely to be an order of magnitude larger than any other ML experiment performed thus far in drug discovery!
Owkin coordinated the winning proposal, now elaborated into the “co-opetitive” (simultaneously cooperative and competitive) MELLODDY platform. The MELLODDY workhorse for collaborative and privacy preserving machine learning is federated learning (FL). In FL, data never leave the infrastructures of any pharma partner. The machine learning process occurs locally at each participating pharmaceutical company and only the models are shared. Thus, the predictive power of the resulting model can benefit from all datasets while protecting the data. Necessarily, the individual information stored within the model must remain private and an important research effort is devoted to guaranteeing that only statistical information is shared between partners. In fact, MELLODDY pushes beyond the privacy requirements of traditional FL, because the pharmaceutical companies are investigating different experimental assays, which must remain private in that they cannot be disclosed to each other.
It is the first platform for multi-task multi-partner learning where the nature of the tasks cannot be shared between partners
Owkin is proud to power this new type of collaboration with its Federated Multi-task Learning platform called Owkin Connect, which is based on the open-source Substra framework. As the coordinator of the project, Owkin organizes contributions from several European startups and academics to build the single technological stack displayed below.
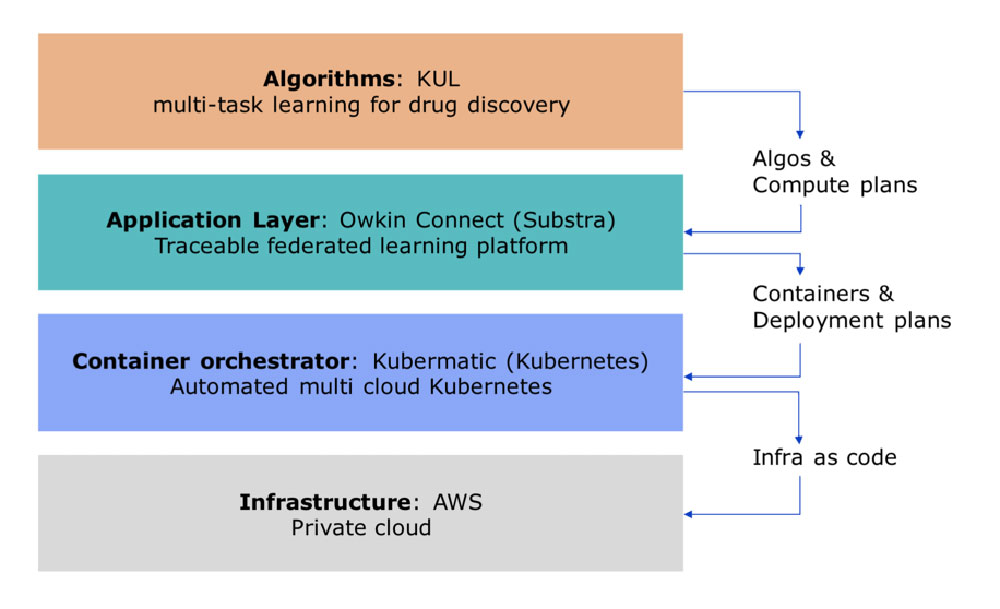
KU Leuven is providing specific and advanced machine learning algorithms for drug discovery that are refactored by NVIDIA for efficiency. Owkin Connect coordinates federated learning runs with these algorithms, as an executive layer on top of a Kubernetes orchestrator; Loodse deploys the infrastructure over an AWS provided private cloud. To support this platform several other actors are participating to perform the associated research underlying this project: Iktos brings along their expertise in drug discovery while BME provides their expertise in privacy of machine learning applied to drug discovery. The Substra Foundation monitors run execution and lead the communication and sustainability of the project.
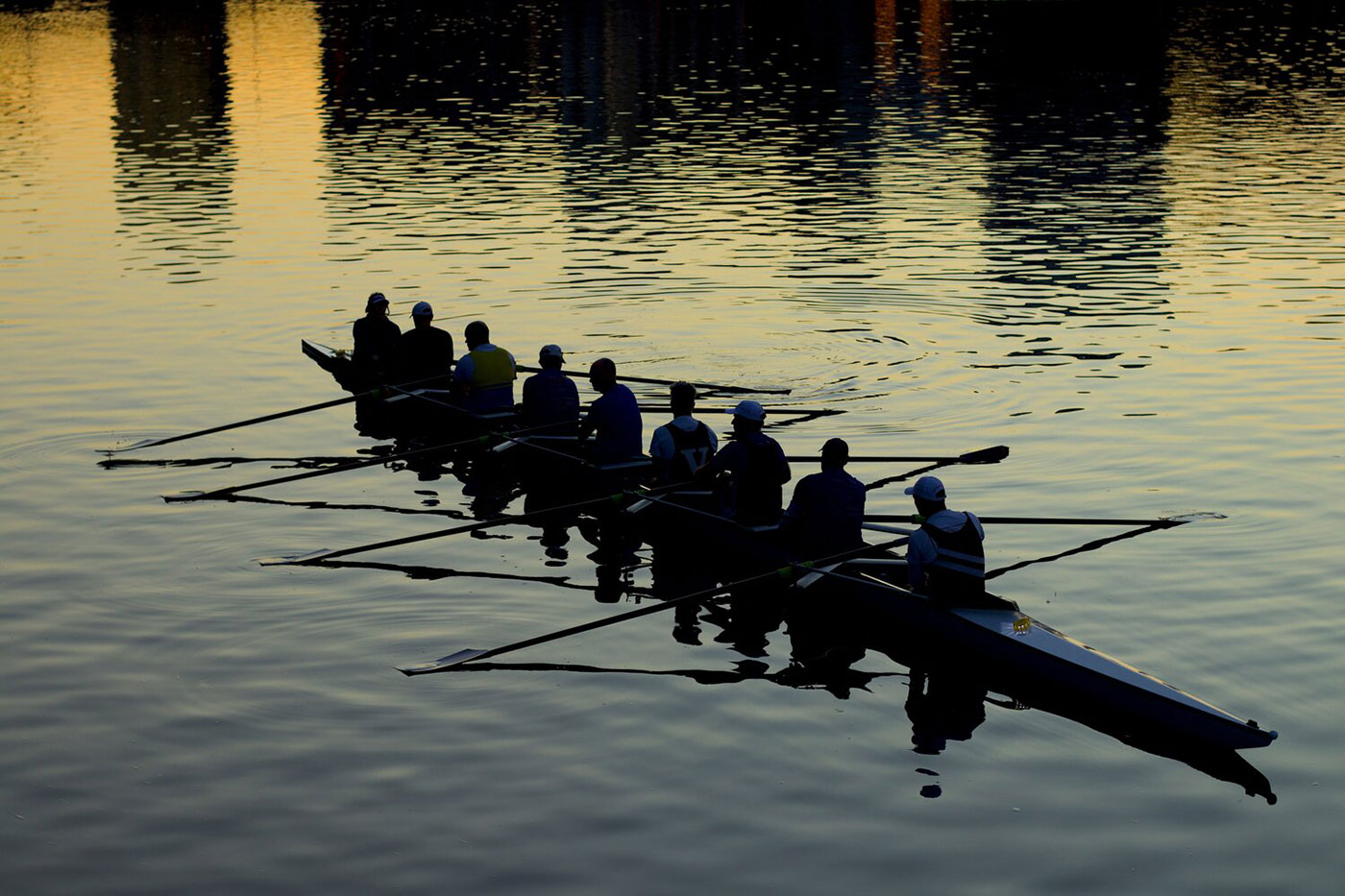
MELLODDY is a challenging project not only from a technical standpoint, but also because it is widely diverse in terms of fields of expertise, culture and backgrounds of those involved in the project. Every day we meet new challenges which can be common in collaborative projects or unexplored territory. At Owkin, we firmly believe that this is how innovation is to be done in the 21st century: welcoming cultural diversity and competition so that the outcomes are more than the sum of its parts.
This initial post will kick off a series of articles authored by the consortium partners respectively, in which they will explore their unique considerations for participating in MELLODDY, the relevance of this work, and the insights we are generating along the way. We’re only just beginning, so stay tuned!
- DiMasi JA et al., 2016. Innovation in the pharmaceutical industry: new estimates of R&D costs. Journal of Health Economics 47, 20-33.
- Scannell, J., Blanckley, A., Boldon, H. et al. Diagnosing the decline in pharmaceutical R&D efficiency. Nat Rev Drug Discov 11, 191–200 (2012) doi:10.1038/nrd3681