Owkin in depth: pushing boundaries in AI research
The human brain is a marvel—86 billion neurons, 100 trillion connections, limitless potential. And yet, even with all that power, fully understanding human biology remains one of our greatest challenges. To crack its complexity, we need to push beyond human limits—we need AI that doesn’t just complement our intelligence, but surpasses it.
This is really hard to do. Owkin is building AI to decode biology at a scale no human brain alone can achieve, accelerating breakthroughs in diagnostics, biomarkers, drug targets, and therapeutics. From uncovering hidden patterns in medical data to powering research co-pilots for scientists, we’re bringing intelligence to the frontier of biomedical discovery.
Over the past year, our Research & Technology team has reached major milestones in advancing generalizable AI for digital pathology, AI-powered spatial transcriptomics, and federated learning for biomedical research, and in prototyping agentic AI co-pilots for scientists.
In this blog:
- Digital pathology
- AI-powered spatial transcriptomics
- Federated learning for biomedical research
- Prototyping agentic AI co-pilots for scientists
Generalizable AI for pathology
In computational pathology, a common task is to analyze digitally scanned tissue samples to predict endpoints that enhance disease understanding and improve patient care. At Owkin, this often involves predicting clinical outcomes such as overall survival (OS) and progression-free survival (PFS), as well as biomarkers like microsatellite instability (MSI/MSS) status. A key requirement for deploying these models in real-world settings is their robustness to variations in slide preparation (e.g., staining) and digitization (e.g., scanner differences), ensuring reliability across different clinical centers.
Recognizing the need for high-performing AI diagnostics, Owkin partnered with MSD to co-develop and commercialize AI-powered digital pathology solutions for multiple cancer indications. This collaboration highlighted the challenge of developing robust AI models for low-prevalence cancers, where available data is scarce. Over the past year, Owkin’s Research & Technology team has made significant progress in addressing these challenges—building robust foundation models and pioneering innovative techniques for model calibration and uncertainty quantification.
Generalizable feature extraction with Foundation Models
State-of-the-art computational pathology pipelines rely on foundation models (FMs)—feature extractors that can scale up to 1 billion parameters and are trained on millions of whole-slide images (WSIs). To advance AI-driven pathology, Owkin compiled a dataset of 460 million pathology tiles spanning more than 30 cancer types, sourcing histopathology slides from over 100 publicly available cohorts.
Using this dataset, our team trained a self-supervised vision transformer-based FM and publicly released the model.
The resulting FM, called Phikon-v2 1,2, achieved performance on par with comparable private models and has been widely adopted by the research community as a standard for digital pathology3,4,5,6,7. Phikon-v2 also demonstrated strong generalizability, performing well across multiple cancer types and tasks—its success culminating in a first-place finish at the UCB-OCEAN Kaggle competition.
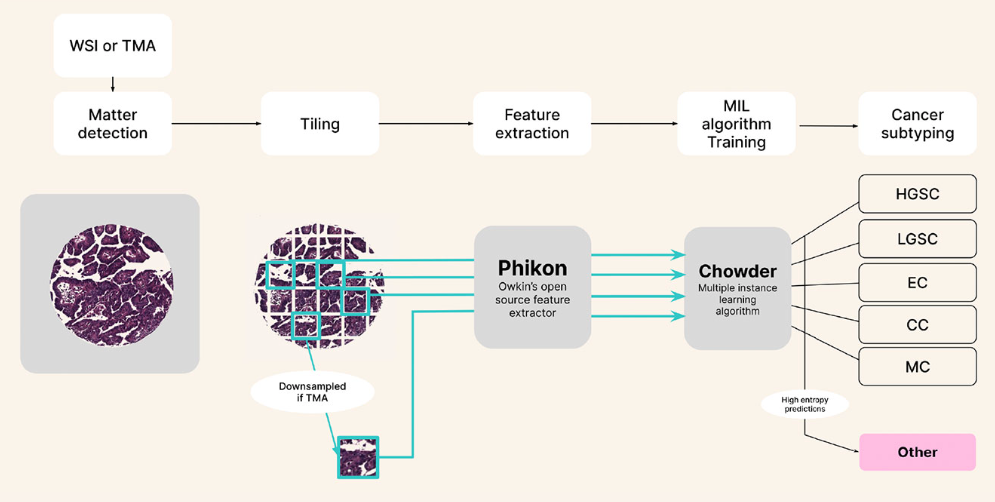
Distillation of foundation models (FM) for digital pathology
The rise of foundation models (FMs) in digital pathology has led to remarkable performance gains, but at the cost of increased computational and inference time. Despite their growing adoption, FM robustness remains an underexplored challenge—yet it is critical for clinical deployment. To address this, Owkin and Bioptimus conducted a study demonstrating that applying distillation techniques to digital pathology FMs significantly enhances robustness while drastically reducing computational costs—without compromising performance8. With the goal of making robust FMs more accessible to researchers with limited computational resources, Owkin and Bioptimus released the distilled model H0-mini alongside a new benchmark, plismbench, designed to evaluate FM robustness in digital pathology. Plismbench leverages PLISM, a multi-scanner, multi-staining public dataset created to assess AI models’ sensitivity to domain shifts caused by variations in scanners and staining protocols.

Uncertainty quantification and model calibration
Despite recent advances towards building robust foundation models (FMs) for digital pathology, perfect generalization remains out of reach. Enhancing model robustness beyond feature extraction is therefore just as crucial—particularly in areas like predictive uncertainty, which assesses a model’s confidence in its own predictions. However, no standardized framework currently exists for evaluating these methods before clinical deployment. To address this, Owkin developed a new uncertainty metric for assessing predictive uncertainty in binary classification and validated it along with an existing metric, in a research paper accepted at AISTATS 20259. These metrics, UQ-AUC and UQ-C-Index, can be computed using standard class labels, making them practical to implement.
.gif)
Another key approach to improving robustness is model calibration, which ensures that AI models produce consistent predictions across different medical contexts. Calibration typically involves adjusting a model’s sensitivity or specificity using a small set of held-out data after training. However, existing methods10,11 often require tens to hundreds of positive samples for calibration—an impractical demand for low-prevalence cancer indications, such as those in Owkin’s partnership with MSD. To overcome this challenge, Owkin’s Research & Technology team developed a novel methodology, Tile Score Matching (TSM)12, which enables precise sensitivity calibration for whole-slide image classification using as few as five positive samples.
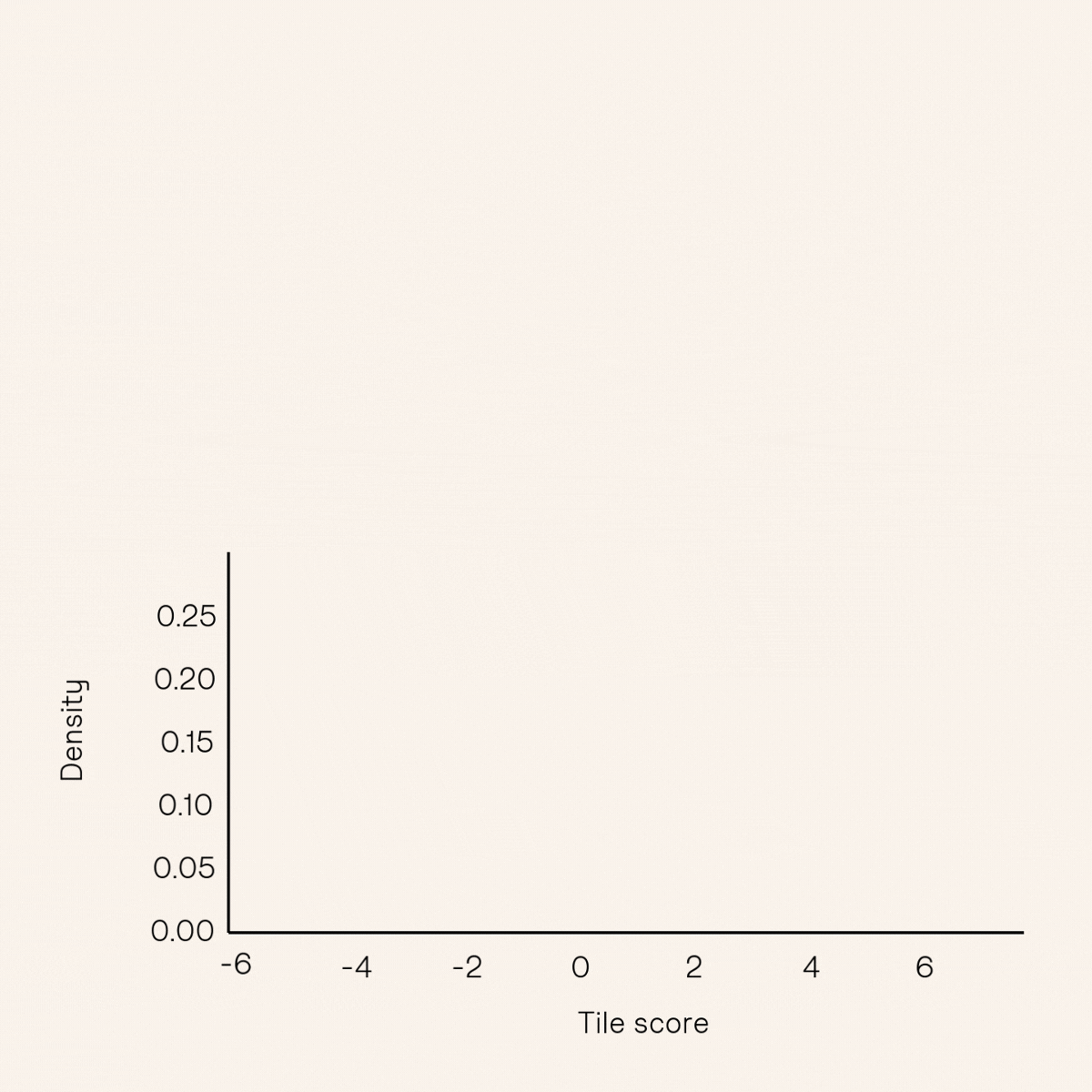
Advancing AI for spatial omics research
Owkin has been at the forefront of spatial omics research through MOSAIC, our flagship spatial omics initiative where we collaborate with five leading cancer research centers to map the tumor microenvironment of thousands of patients with unprecedented resolution. By integrating 6 data modalities including bulk, single-cell and spatial transcriptomics, digital pathology, whole-exome sequencing and clinical information, we are uncovering how cellular interactions drive disease progression and treatment response. Through a series of projects, we have explored how AI methodologies can enable deeper insights from tumor microenvironment analysis by overcoming challenges related to spatial context, resolution, and reproducibility.
Leveraging spatial patterns in digital pathology models
Traditional multiple instance learning (MIL) approaches in digital pathology often treat image patches as independent and overlook spatial dependencies. To address this limitation, we developed Context-Aware Regularization on Multiple Instance Learning (CARMIL)13. By incorporating spatial regularization, CARMIL ensures that biologically relevant features—such as cancer cell clustering or tumor margins—inform the model’s predictions.
CARMIL’s effectiveness was validated through survival analysis in glioblastoma (TCGA GBM) and colorectal cancer (TCGA COAD), where it significantly improved the interpretability and accuracy of prognostic models. Compared to baseline MIL methods, CARMIL boosted the concordance index (C-index) by 15% for TCGA GBM and 18% for TCGA COAD. Furthermore, patients stratified by CARMIL predictions showed significant differences in overall survival (OS) (p < 0.01), underscoring its robustness.

Learning to master spatial transcriptomics technologies
Spatial biology is a rapidly evolving field, with technologies for spatially resolved gene expression advancing at an unprecedented pace. These methods generate vast amounts of valuable data, but extracting meaningful insights requires a deep understanding of the sequencing technologies, their inherent biases, and potential artifacts.
To address this, researchers from Owkin and Lausanne University Hospital conducted a study comparing three methods14 for analyzing gene expression in archived tumor samples: GeoMx DSP, Visium CytAssist, and Chromium Flex. The study assessed each method’s operational efficiency, cell-type signature specificity, and ability to capture tumor heterogeneity.
The study found that all three methods produced high-quality, highly reproducible transcriptomic data from serial sections of the same FFPE block. Both GeoMx and Visium demonstrated the ability to enrich rare cell type signatures. From an operational standpoint, GeoMx required more resources to set up and run and was more susceptible to batch effects but offered greater flexibility in experimental design. Chromium, on the other hand, had lower running costs but required more tissue to conduct experiments.
Overall considering the results, Visium and Chromium were recommended for high-throughput and discovery projects due to their unbiased profiling and strong ability to capture tumor heterogeneity, whereas GeoMx was deemed more suitable for targeted experiments to address specialised questions. The study also underscored the importance of integrating matched single-cell RNA sequencing (scRNA-seq) data to fully account for cellular heterogeneity.
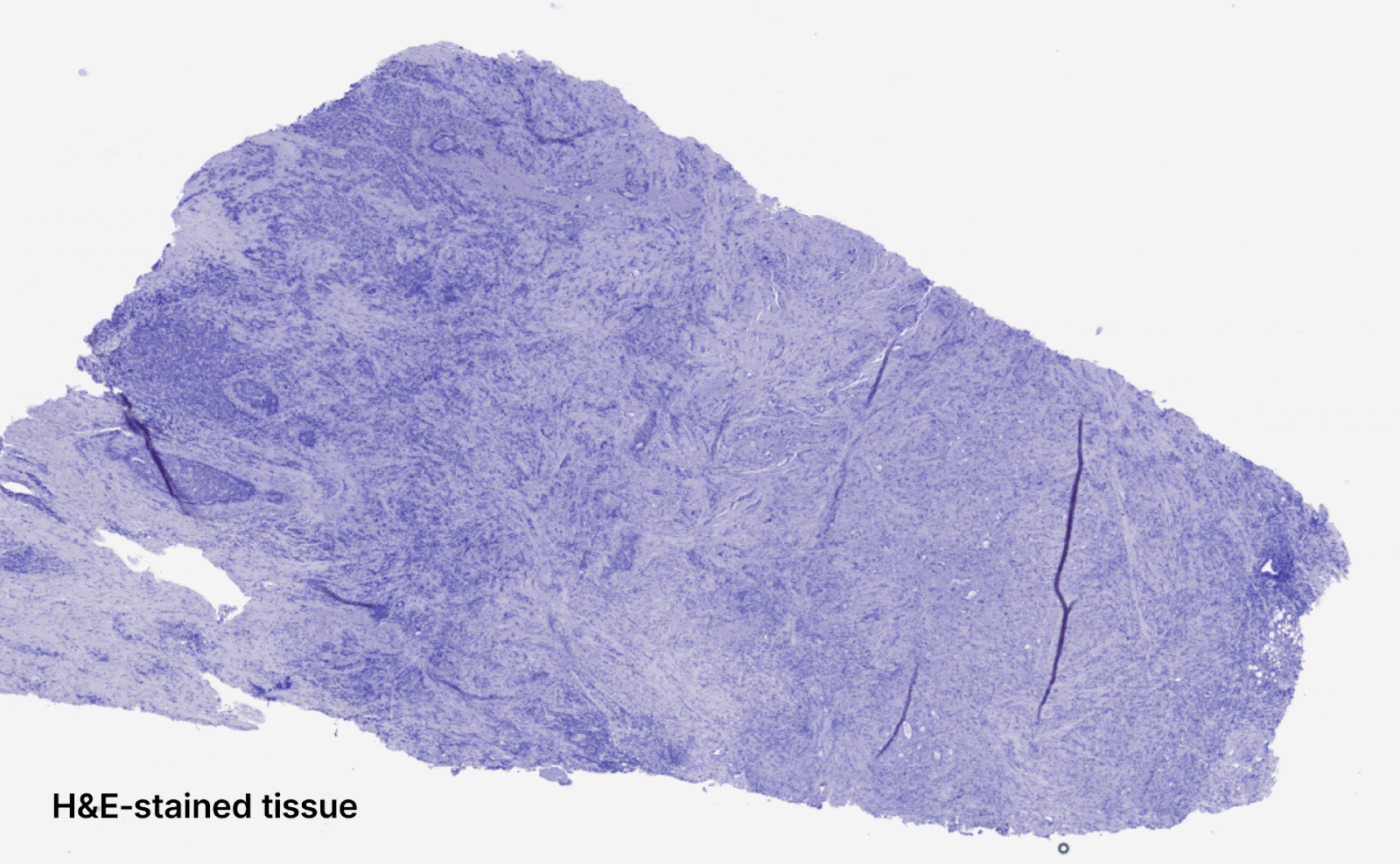
Predicting spatialized gene expression from H&E slides
To bridge the gap between routine H&E slides and spatial transcriptomics (spTx), we developed Multiscale Integration of Spatial Omics (MISO)15. Trained on a novel dataset of 72 spatial transcriptomics samples from 10x Genomics Visium, MISO can predict spatial gene expression at near single-cell resolution directly from digitized H&E slides. This unlocks downstream applications such as prediction of biomarkers like Microsatellite Instability (MSI) and molecular phenotyping, offering a cost-effective and scalable alternative to generating spatial transcriptomics data from patient samples.
In validation studies, MISO achieved a Pearson correlation coefficient of 0.65–0.75 for spatial gene expression across multiple genes, demonstrating strong predictive accuracy. The model also consistently identified clinically relevant spatial gene patterns, enriching insights into tumor microenvironment heterogeneity. By establishing an upper-bound estimate for spatial gene prediction performance, MISO paves the way for large-scale integration of histopathological and molecular data.

Quantifying cellular composition from H&E slides
Despite major advancements in foundation models for pathology and the maturity of detection architectures, developing a universal tool for accurate cell detection, segmentation, and classification remains a challenge. A key limitation is the scarcity of comprehensive, high-quality annotated training datasets.
To address this, we constructed an unprecedented large-scale pan-cancer dataset encompassing major cell types, including rare ones. Using this dataset, we developed state-of-the-art detection models to create an AI tool capable of simultaneously detecting, segmenting, and classifying all major cell types across diverse cancer types16. Specifically, we are developing a series of models called HIPE (Histo Interpretability Prediction Engine), designed to identify key histological structures, including cell types (e.g., normal, tumor, immune cells), tissue types (e.g., fibrosis), and critical morphological features (e.g., lymphovascular invasion, necrosis, perineural invasion). These models have been integrated into our AI pipeline and are now systematically applied across all our projects that feature histology datasets.

Federated learning for biomedical research
Federated Learning (FL) allows institutions with sensitive data to collaboratively train machine learning (ML) models at scale without sharing raw data. This is especially valuable in healthcare, where patient confidentiality and proprietary data protection are paramount. In FL, only the models move between sites, while the data remains securely stored locally. However, the adoption of FL in biomedical research has been limited by the lack of dedicated methodologies. To address this, Owkin has developed two FL approaches specifically designed for biomedical research: one for causal inference and another for RNA sequencing (RNA-Seq) data analysis.
Federated External Control Arms (FedECA)
The clinical development of new drugs faces significant challenges, including high failure rates in Phase III trials. One way to mitigate this is by incorporating real-world data through an external control arm (ECA) analysis in a "Phase 2.5," providing early evidence of a drug’s efficacy and potentially preventing costly failures before Phase III. However, privacy regulations make accessing diverse, representative datasets for ECAs particularly challenging.
FL offers a solution by allowing researchers to analyze data across institutions while ensuring that sensitive patient information remains local. Owkin scientists have developed a novel approach, FedECA17, which combines FL with ECA techniques to analyze distributed outcomes while minimizing patient data exposure. The feasibility of FedECA was demonstrated through experiments on both simulated and real-world datasets using Substra, an open-source FL platform designed for privacy-sensitive healthcare applications. To foster collaboration and transparency, the code for this analysis has been publicly released, enabling the broader scientific community to build upon this work.
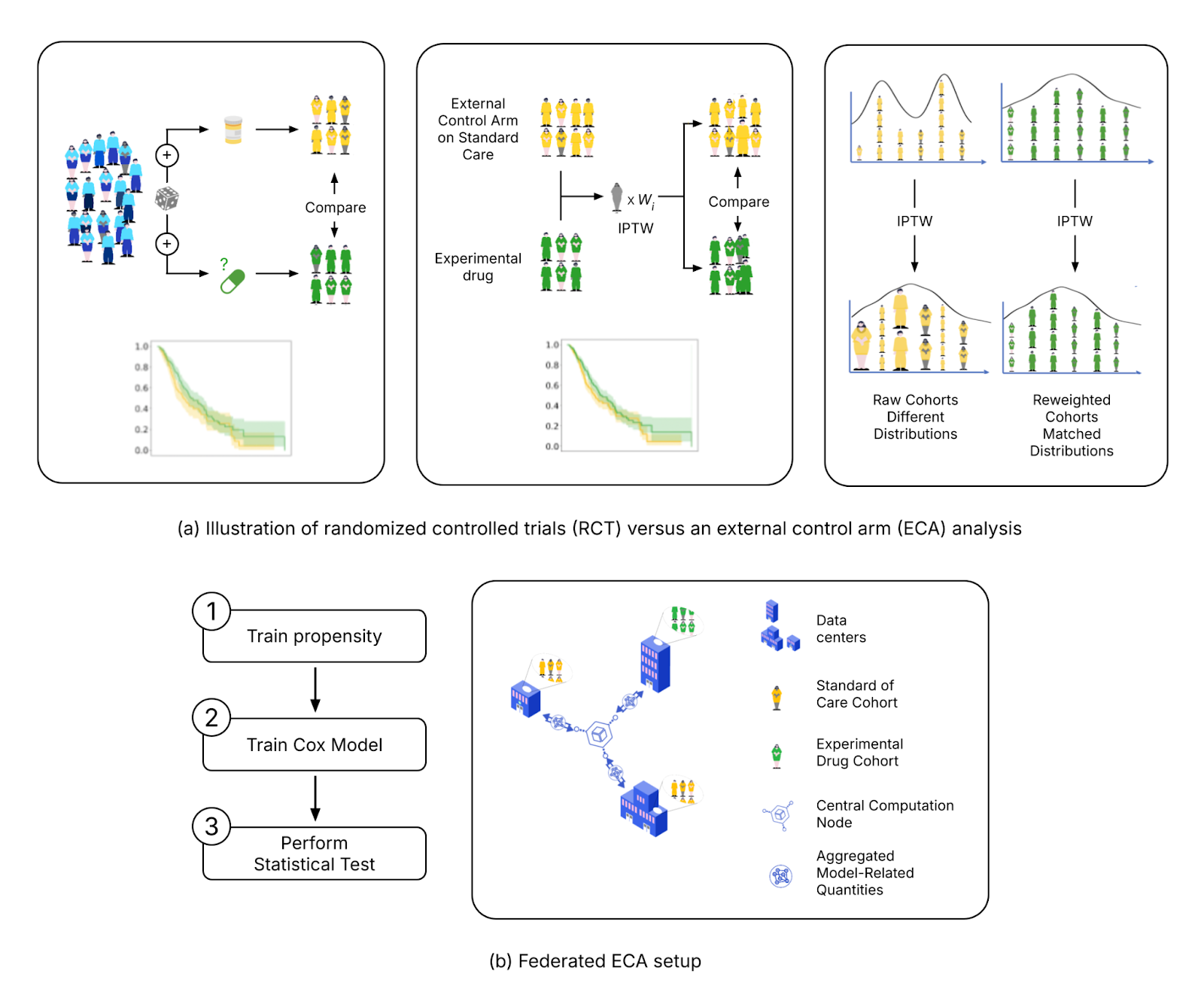
FedPyDESeq2 - Differential Expression Analysis (DEA) in federated learning
Differential Expression Analysis (DEA) is a cornerstone of cancer research and a primary application of RNA sequencing (RNA-seq). However, due to legal and privacy constraints, DEA is often limited by data silos, limiting the scale and depth of DEA studies. This fragmentation can hinder clinical insights and slow down the discovery of new treatment opportunities. To overcome these barriers and enable large-scale transcriptome studies, Owkin recently developed FedPyDESeq218, a federated software for DEA on siloed bulk RNA-seq data. Built on Substra, FedPyDESeq2 implements the DESeq219 pipeline while preserving data privacy.
FedPyDESeq2 was benchmarked on eight different TCGA cancer indications, with datasets split into silos based on geographical origin. It achieved near-identical results compared to PyDESeq220 on pooled data. Moreover, in scenarios with high data heterogeneity, FedPyDESeq2 outperformed state-of-the-art meta-analysis approaches, achieving nearly 100% precision and 93% recall—whereas the best existing methods reached only 80% precision and 60% recall, with performance degrading further as data heterogeneity increased. By combining privacy-preserving computation with cutting-edge transcriptomic analysis, FedPyDESeq2 paves the way for more robust, scalable, and collaborative RNA-seq research.
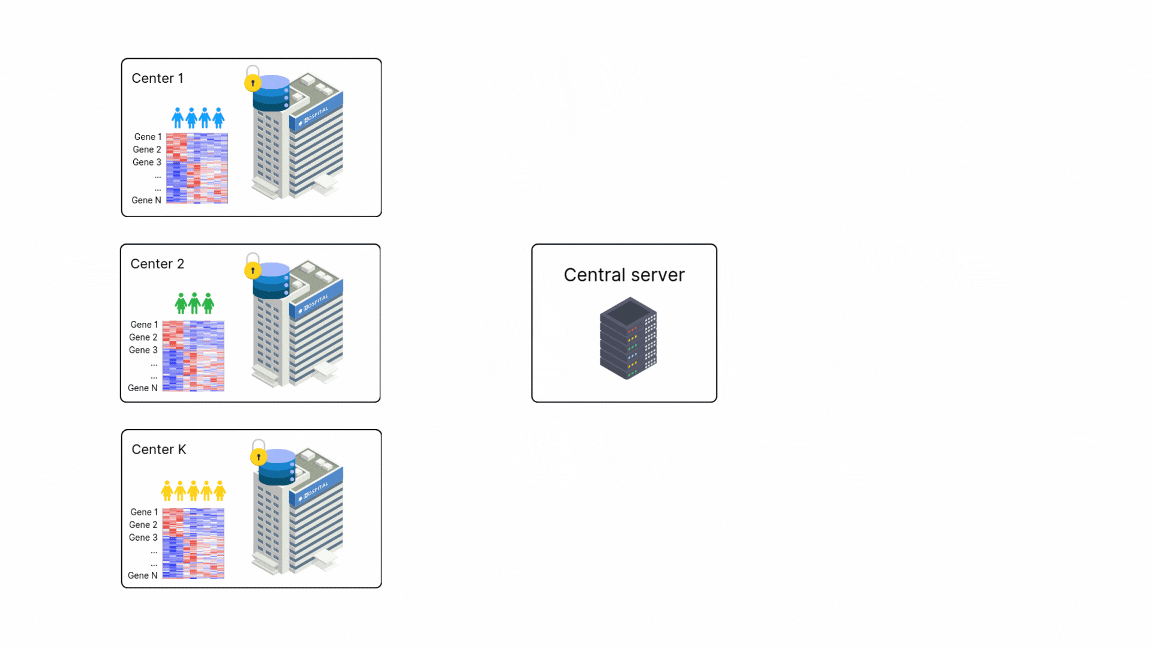
Prototyping co-pilot agentic AI tools
Recent advancements in AI agents and LLM-powered productivity tools, such as coding copilots, have transformed several industries. However, the development of efficient LLM tools trained on specialized biological content to assist biologists in critical tasks like literature review and data analysis is still in its infancy. To address this gap, Owkin has developed AI-driven copilot tools tailored for biomedical experts and AI scientists.
Looking ahead: The development of Owkin K, an agentic AI-based research co-pilot
In the last 12 months we published over 20 research articles (peer-reviewed and preprints), demonstrating how Owkin is staying at the cutting edge of methodological AI research, making remarkable progress in generalizable pathology models, AI for spatial transcriptomics, FL for biomedical research, and the development of co-pilot tools for scientists based on agentic AI and LLMs. In a technological landscape that continues to evolve at an incredibly fast pace, we are presented with exciting scientific opportunities. Above all, our Research & Technology team is developing K Navigator, an agentic AI-based research co-pilot that can autonomously access and interpret comprehensive scientific literature, large-scale biomedical data, and tap into the power of Owkin’s AI for biological discovery. In their first iteration K Navigator will be capable of understanding complex biological queries and synthesizing knowledge from across the scientific literature and 30+ databases (ChEMBL, TCGA, Open Targets, GTEx, etc) to provide scientific summaries and insights to researchers. K Navigator will be able to iterative explore TCGA and MOSAIC Window data through analysis and visualization in response to user prompts.
Building on K Navigator, the team is developing K Pro, a version of the co-pilot designed to tackle complex pharmaceutical challenges across data exploration, drug discovery, and drug development. In the future these AI agents will become more sophisticated and will be able to plan and execute experiments in fully automated, robotized wet labs, where real-world human biology is simulated with patient-derived organoids. The results of these experiments will feed back into the system, enabling continuous learning and refinement of the AI agents’ models.
As AI research accelerates at an unprecedented pace, we remain committed to pushing the boundaries of innovation while contributing to the broader AI and ML research community. From advancing generalizable AI in pathology to prototyping research co-pilots that navigate the ever-expanding scientific landscape, our work not only drives real-world impact in healthcare but also helps shape the future of AI-driven discovery.
Bibliography
1 A. Filiot et al., “Scaling Self-Supervised Learning for Histopathology with Masked Image Modeling,” Pathology, preprint, Jul. 2023. doi: 10.1101/2023.07.21.23292757.
2 A. Filiot, P. Jacob, A. Mac Kain, and C. Saillard, “Phikon-v2, A large and public feature extractor for biomarker prediction,” 2024, arXiv. doi: 10.48550/ARXIV.2409.09173.
3 J. Breen, K. Allen, K. Zucker, L. Godson, N. M. Orsi, and N. Ravikumar, “A comprehensive evaluation of histopathology foundation models for ovarian cancer subtype classification,” Npj Precis. Oncol., vol. 9, no. 1, p. 33, Jan. 2025, doi: 10.1038/s41698-025-00799-8.
4 D. Ferber et al., “In-context learning enables multimodal large language models to classify cancer pathology images,” Nat. Commun., vol. 15, no. 1, p. 10104, Nov. 2024, doi: 10.1038/s41467-024-51465-9.
5 X. Ji et al., “Physical Color Calibration of Digital Pathology Scanners for Robust Artificial Intelligence–Assisted Cancer Diagnosis,” Mod. Pathol., vol. 38, no. 5, p. 100715, May 2025, doi: 10.1016/j.modpat.2025.100715.
6 M. Mallya, A. K. Mirabadi, D. Farnell, H. Farahani, and A. Bashashati, “Benchmarking histopathology foundation models for ovarian cancer bevacizumab treatment response prediction from whole slide images,” Discov. Oncol., vol. 16, no. 1, p. 196, Feb. 2025, doi: 10.1007/s12672-025-01973-x.
7 R. Wang et al., “Deep learning for predicting prognostic consensus molecular subtypes in cervical cancer from histology images,” Npj Precis. Oncol., vol. 9, no. 1, p. 11, Jan. 2025, doi: 10.1038/s41698-024-00778-5.
8 A. Filiot et al., “Distilling foundation models for robust and efficient models in digital pathology,” 2025, arXiv. doi: 10.48550/ARXIV.2501.16239.
9 A. Pignet, C. Regniez, and J. Klein, “Legitimate ground-truth-free metrics for deep uncertainty classification scoring,” arXiv. doi: 10.48550/ARXIV.2410.23046
10 C. Saillard et al., “Validation of MSIntuit as an AI-based pre-screening tool for MSI detection from colorectal cancer histology slides,” Nat. Commun., vol. 14, no. 1, p. 6695, Nov. 2023, doi: 10.1038/s41467-023-42453-6.
11 M. Roschewitz et al., “Automatic correction of performance drift under acquisition shift in medical image classification,” Nat. Commun., vol. 14, no. 1, p. 6608, Oct. 2023, doi: 10.1038/s41467-023-42396-y.
12 A. Pignet et al., “Robust sensitivity control in digital pathology via tile score distribution matching,“ arXiv. doi: 10.48550/ARXIV.2502.20144
13 T. Nait Saada, et al., “CARMIL: Context-Aware Regularization on Multiple Instance Learning models for Whole Slide Images,” Proceedings of the MICCAI Workshop on Computational Pathology, PMLR 254:154-169, 2024.
14 Y. Dong, et al., “Transcriptome Analysis of Archived Tumor Tissues by Visium, GeoMx DSP, and Chromium Methods Reveals Inter- and Intra-Patient Heterogeneity” bioRxiv. doi: 10.1101/2024.11.01.621259
15 B. Schmauch et al., “A deep learning-based multiscale integration of spatial omics with tumor morphology,” bioRxiv. doi: 10.1101/2024.07.22.604083
16 L. Gillet “AI for histology-based spatial biomarker discovery” Presentation at BioTechX Europe, 9-10 October 2024.
17 J. O. du Terrail et al., “FedECA: A Federated External Control Arm Method for Causal Inference with Time-To-Event Data in Distributed Settings,” 2023, arXiv. doi: 10.48550/ARXIV.2311.16984.
18 B. Muzellec, U. Marteau-Ferey, and T. Marchand, “FedPyDESeq2: a federated framework for bulk RNA-seq differential expression analysis,” Dec. 10, 2024, Bioinformatics. doi: 10.1101/2024.12.06.627138.
19 Love MI, Huber W, Anders S. Moderated estimation of fold change and dispersion for RNA-seq data with DESeq2. Genome Biol 2014;15:550.
20 Boris Muzellec, Maria Teleńczuk, Vincent Cabeli, Mathieu Andreux, PyDESeq2: a python package for bulk RNA-seq differential expression analysis, Bioinformatics, Volume 39, Issue 9, September 2023, btad547, https://doi.org/10.1093/bioinformatics/btad547