Leveraging machine learning to optimize clinical trials
Key Messages
- Covariate adjustment is a method to reduce sample size or increase statistical power in clinical trials;
- It leverages meaningful clinical patient characteristics, including risk scores;
- Machine learning (‘ML’) can improve the predictive accuracy of these risk scores; and
- ML, therefore, allows for more efficient clinical trials.
Introduction
The achievement of sufficient statistical power to prove a therapeutic effect requires the recruitment of large numbers of patients. This fact is reflected in the great cost and long duration historically associated with clinical trial programs.
However, there is another more effective way to achieve sufficient power beyond increasing the sample size. We can reach this improvement in power by adjusting the efficacy analysis on covariates associated with the endpoint of a clinical trial. This approach mitigates much of the variance that would otherwise obscure the treatment effect, reducing the noise, so the signal stands out.
For example, age is a well-known predictor of Covid-19 severity. Taking this factor into account in ongoing clinical trials would allow for better estimates of treatment effect.
In this article we will explain the concept of covariate adjustment, and its potential impact on clinical development.
What is Covariate Adjustment?
You can understand Covariate adjustment using a metaphor regarding signal and noise.
To illustrate this, imagine you are on the subway and trying to listen to music like in the cover image above! The noise of the subway drowns out the music coming from your headphones. One solution could be to increase the volume, and risk long-term damage to your hearing. But a better alternative would be to use a noise-canceling headset. This would then compensate for the noise of the subway, allowing you to listen to music at a safe volume.
In a clinical trial, the signal (ie, the music) we want to listen to is the treatment effect. The noise of the subway is the variability in treatment outcomes that can be explained by covariate factors, such as medical history. To better “hear” the signal, we must either increase the volume (ie, the sample size) or cancel out the noise (adjust for covariates that are predictive of the endpoint, thereby reducing the heterogeneity in treatment outcomes not explained by treatment effect).
Covariate adjustment effectiveness is well documented in clinical trial methodology literature1, and accepted by regulators. In particular, the European Medicines Agency (‘EMA’) Guideline on adjustment for baseline covariates in clinical trials states:
The main reason to include a covariate in the analysis of a trial is evidence of a strong or moderate association between the covariate and the primary outcome measure. Adjustment for such covariates generally improves the efficiency of the analysis and hence produces stronger and more precise evidence (smaller p-values and narrower confidence intervals) of an effect.2
As covariate adjustment allows researchers to capture the same information with a smaller sample size, it presents an opportunity to run more efficient trials aligned with regulatory standards.
How can Covariate Adjustment impact clinical trials?
New technologies, such as advances in genomics and artificial intelligence, may have a rapid and positive impact on clinical development by supplying new predictive covariates. For example, the establishment of polygenic risk scores (risk scores based on your genetics), while still ongoing, may eventually be of use in trials for the prevention of cardiovascular disease3. Similarly, deep convolutional neural networks have already revolutionized computer vision. For instance, such networks have applications in imaging data across medicine, most notably in radiology, ophthalmology, dermatology, and histopathology4. Using these techniques enables researchers to capture previously unexplained heterogeneity in prognosis. In fact, the application of our tailored deep learning algorithm has already been shown to improve the prediction of overall survival vs. the current pathologist-led actual predictions in mesothelioma5 (a lung cancer predominantly caused by asbestos).
Mesothelioma Use Case
At Owkin, we developed MesoNet, a machine learning model that predicts the overall survival of malignant mesothelioma patients using whole slide (histology) images of tumor tissue. These new survival predictions are better than the existing subtype classification utilized by pathologists. Therefore, leveraging MesoNet can reduce the sample size of mesothelioma trials, lowering costs, and reducing trial lengths. In fact, we estimate that MesoNet’s deep learning approach compared to standard subtyping would allow researchers to reduce the sample size requirements of three, large phase 3 clinical trials in mesothelioma by 6-13%, resulting in millions of dollars of savings. Furthermore, assuming that patient enrollment is conducted on a rolling basis, the decrease in sample size obtained using MesoNet would allow for a 2-8 month reduction in trial duration vs current practice.
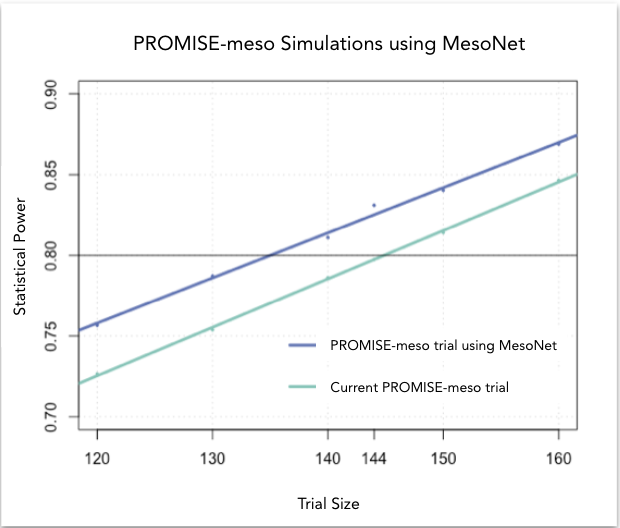


Conclusion
In conclusion, covariate adjustment powered by machine learning stands to fundamentally change the way clinical trials are conducted. By systematically adjusting predictions for well-established covariates, we can accelerate clinical development programs and reduce costs — and do so at a scale that will bring real progress.
At Owkin, we are currently collaborating with regulators to set the foundations of machine learning-powered clinical trials. However, optimizing trial protocols is just the beginning — machine learning will make a lasting impact on other steps of the clinical development pipeline as well. This impact is visible after early-stage clinical trials. In cases such as these, a pharmaceutical company must decide, based on minimal data, whether the drug efficacy is promising enough to start a long and expensive phase 3 trial. Stay tuned – This will be the topic of another post.
References:
- Kahan, B. C., Jairath, V., Doré, C. J. & Morris, T. P. The risks and rewards of covariate adjustment in randomized trials: an assessment of 12 outcomes from 8 studies. Trials 15, 139 (2014).
- Committee for Medicinal Products for Human Use. Guideline on adjustment for baseline covariates in clinical trials. EMA (2015).
- Inouye, M. et al. Genomic Risk Prediction of Coronary Artery Disease in 480,000 Adults: Implications for Primary Prevention. J. Am. Coll. Cardiol. 72, 1883–1893 (2018).
- Esteva, A. et al. A guide to deep learning in healthcare. Nat. Med. 25, 24–29 (2019).
- Courtiol, P. et al. Deep learning-based classification of mesothelioma improves the prediction of patient outcome. Nat. Med. 25, 1519–1525 (2019).