Interview with Charlie Saillard - Validating an AI diagnostic
We sat down with Charlie Saillard, Lead Data Scientist at Owkin and first author of the MSIntuit® CRC paper recently published by Nature Communications. With Charlie, we talked about the initial stages of the project, as well as the hidden challenges that the team had to overcome and the breakthroughs that led to a major leap in the performance of the model.
What is your background and what was your contribution to the study as the first author?
My background is purely mathematics. In this study I did the programming part - the actual creation of the model - as well as the performance assessment of the model, which involves a lot of statistics. Some of my co-authors are biologists and pathologists, and during the 3-4 years I worked on this project - we started around 2018 - it was fantastic for me to learn from them about microsatellite instability and the underlying biology of cancer. It was an interesting journey for me, not only learning about the biology side of things, but also being able to witness how to go from a scientific question to an AI model, and to then turn the model into something that can be useful in everyday clinical practice.
What was the idea behind this project?
The overall idea behind this project was to assess whether we could use AI to help give the right treatments to patients with colorectal cancer. And it turns out that patients with a specific phenotype called microsatellite instability [also known as MSI], respond to immunotherapy. So, with this knowledge we set out to develop a model that was able identify these patients directly from histology slides. The idea is that the deployment of such a model in clinical practice could help identify these patients faster and at a lower cost versus using traditional laboratory tests, and ultimately aid clinicians in the correct treatment choice for patients with this kind of cancer.
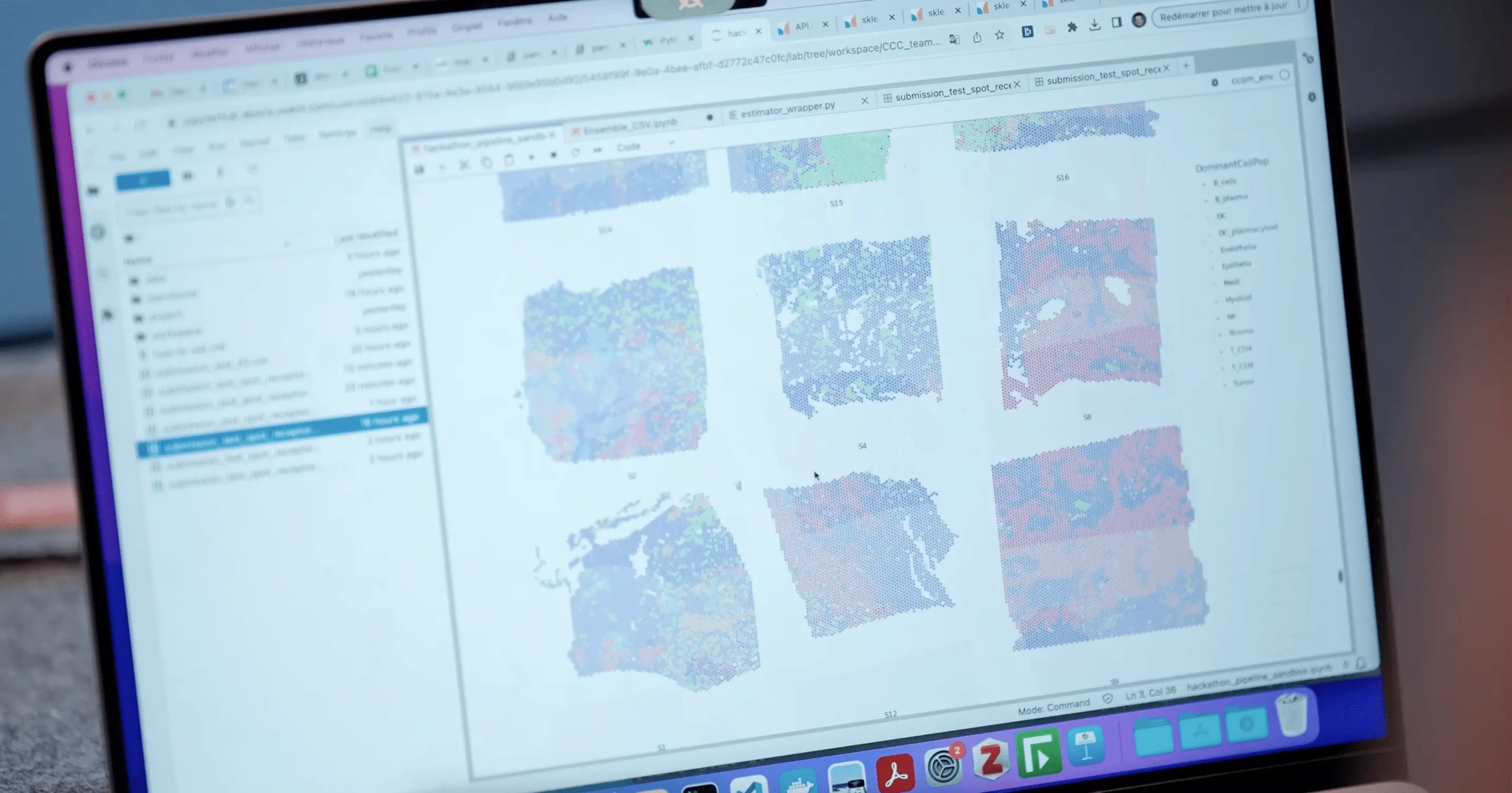
What was the biggest challenge you faced during?
We initially had a promising model with around 80 percent AUC [Area Under the ROC Curve, a graphical plot that illustrates the performance of a binary classifier model] - which was basically very much in line with what another academic group had achieved in a prior publication. That was a great proof of concept and it could have probably been enough for a scientific publication, but it wasn’t good enough to be translated into something usable in clinical practice - you would have too many errors, it wouldn't be useful for physicians. And so we wanted to go beyond that and continued our work, aiming to further enhance the model's performance.
Was there a breakthrough moment during the project that made you overcome this challenge?
Yes. At the time we were using an ML technique called transfer learning, which involves using a model pre-trained on what we call natural images - like animals or objects - only with marginal success. We then came to a breakthrough when we explored self-supervised learning, which resulted in a significantly improved model. At first I couldn’t believe it but it was only after my colleagues were able to reproduce my results that I started being really excited. This is where we began the work to transform our work into a diagnostic tool. At that point I presented our self-supervised learning results at the 24th International Conference on Medical Image Computing & Computer Assisted Intervention in September 2021, where our results received a lot of attention. Two years later, it’s fantastic to see this published in Nature Communications.

What were your biggest worries about the project when you started learning how the model was working?
My primary concern revolved around the uncertainty of the model’s ability to generalize to external data. We consistently questioned whether the strong performance observed on our training sets would hold up when applied to external datasets. As a data scientist, the most fundamental fear is the possibility of your meticulously trained model failing to perform when faced with new data. Several pathologists analyzed the model's most predictive regions and confirmed that the model was highlighting biologically meaningful regions and not capturing bias. We also validated the model on some external data before performing the blind validation and the model performances held. These findings made us confident that the model was robust and that the blind validation would be successful.
What was the most exciting moment during the project?
The most exciting moment has to be when we started to see the tremendous improvement of performance as a result of using self supervised learning. That was very exciting for two reasons: firstly, after several months of marginal improvements using transfer learning, the big jump in model performance almost happened overnight; secondly, as far as I'm aware, it was one of the first proof points that self supervised learning can really help in a task for histology, like MSI prediction. Everyone in the team was very excited about this!
Can you talk about the collaborative aspect and the various moving parts of the project?
Of course. There were lots of moving parts in this project that the team had to take into account beyond the development of the model. To turn this research model into a product that can help physicians in their daily practice, we (the data scientists involved in this project) have been working very closely with the MSIntuit®TM CRC product team at Owkin. We also worked with our collaborators from Medipath to put together a dataset that could answer key questions for the clinical use of the tool, like “was the model robust enough to be used on slides coming from different slide scanners?”. Another important point to mention, which is also the beauty of this kind of AI models, is interpretability: we collaborated with expert pathologists like Magali Svrcek at AP-HP to review the regions of the tissues that the model deemed as being the most predictive, to better understand what histology features the model was finding on the slides. This was extremely useful and it helped us gain even more confidence that the model was using biologically relevant features.
What is the impact of this work?
The model we developed can effectively be used in clinical settings as a pre-screening tool. This may have a direct impact on oncologist decision-making to help bring the best treatment to patients sooner. As a data scientist, it is very exciting when your work has the potential to deliver such benefits.