Blog 4: From AI Model to Validated Medical Device
We are halfway through our Artificial Intelligence in Pathology blog series. So far, we've explored AI's opportunities, the critical importance of data, and the process of building AI models. In this article, we get into why validating these tools is a pivotal step—not only to build trust among pathologists but also as a vital quality assurance for patients, ensuring accurate and reliable diagnoses in real-world settings.
An AI medical device is a regulated, healthcare-specific application of AI designed to support medical decision-making and patient care. This regulation, conducted by organizations such as the European Medicines Agency (EMA) and the US Food and Drug Administration (FDA), ensures patient safety and efficacy. The key component in any regulatory process is validation.
Validation ensures that the model's predictions are accurate, reliable, and generalizable to new, real-world patient samples. It assesses the model's ability to avoid overfitting, where the model might perform well on training data but poorly on new, unseen data. Validation determines whether the model can be trusted for its intended application in a medical device.

Validating the AI model
In the world of machine learning, there are many methods to validate AI models. The general approach to validation in machine learning involves techniques such as cross-validation, which is primarily used in the early stages of development to establish the feasibility of the model's concept. During the development phase, cross-validation and external test cohorts are used to iteratively improve the model's performance. And finally, external clinical validation is conducted as a one-shot process to ensure unbiased evaluation in a real-world clinical setting. When it comes to converting these models into medical devices, you need to ensure that these methods are sufficient to guarantee safety, security, efficacy, and generalizability.
Validating the AI model, therefore, requires a comprehensive approach that takes into account these variabilities, ideally from the outset of medical device development. For example, for our MSIntuitⓇ CRC model, we performed a blind clinical validation on a large external cohort. The validation process included various elements to ensure its generalizability. We also address key questions for use in clinical routine by studying MSIntuitⓇ CRC’s intra and inter-scanner variability, as well as inter-tumor block variability. This comprehensive approach sets Owkin apart, ensuring that our models are not only accurate but also reliable and safe for clinical use.
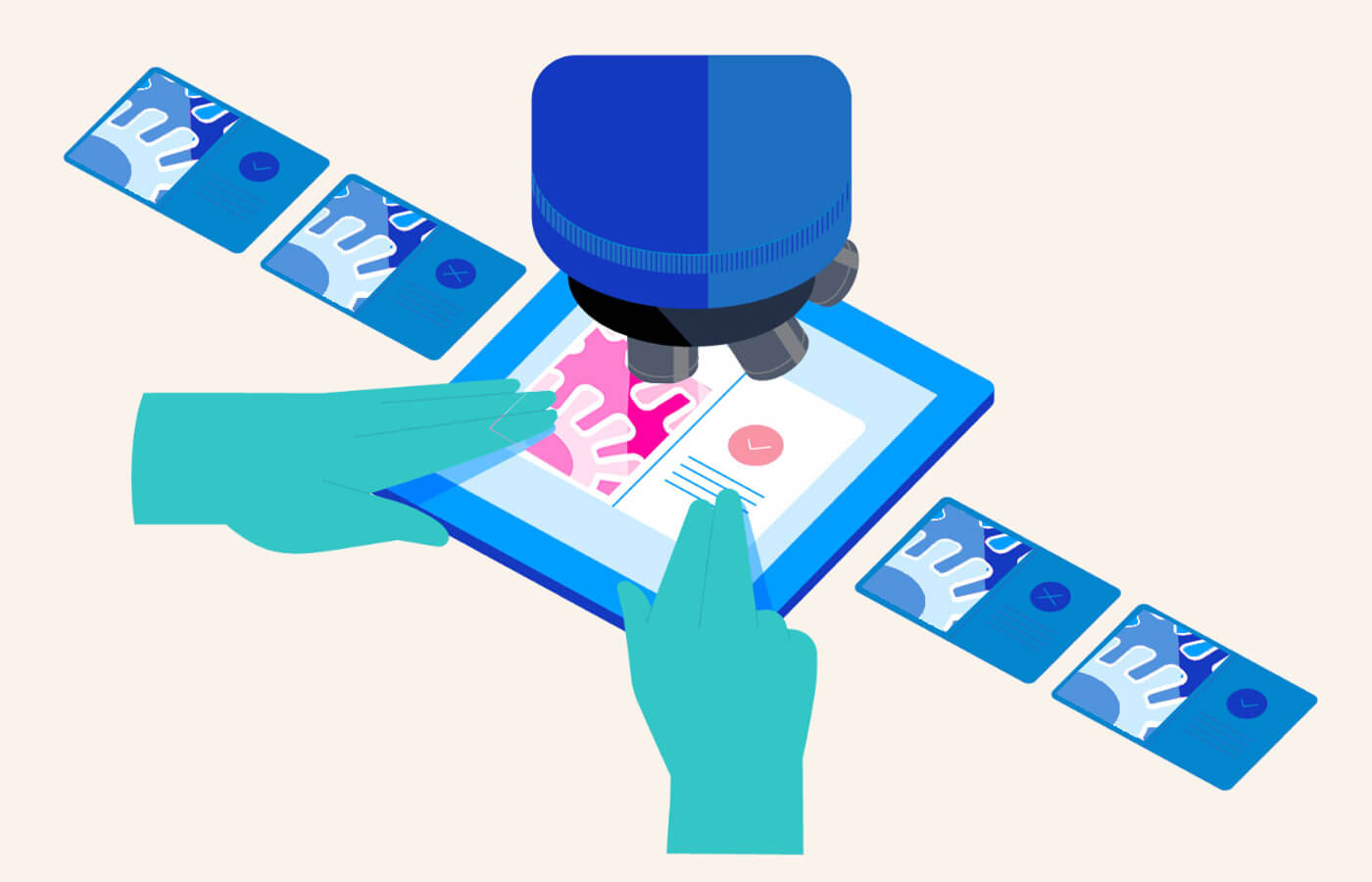
While the exact steps for validation may vary for different AI diagnostic tools, the key component is understanding the potential variations between training and real-world data and validating that these variations do not degrade model performance. This is also the point at which the AI model-turned-medical device is submitted to regulatory bodies for approval, ensuring it meets all necessary standards for safety and efficacy. We'll explore this essential phase of regulatory submission in our next blog.
Continuous monitoring
Validation is designed to identify potential risks before an AI medical device is deployed. However, they are also continuously monitored after deployment to ensure their performance remains accurate, safe, and effective in real-world clinical settings. This involves tracking the model’s output, auditing data quality, gathering user feedback, and reporting errors. Direct and indirect users might provide feedback to identify potential issues, and regular performance assessments help detect any degradation in the model's ability to make correct predictions or any other errors associated with the use of the medical device. Continuous monitoring is essential for patient safety and user experience, as it helps prevent errors, model degradation, and biases that could harm patients.
Building trust in the medical community
Throughout the model development and validation process, engineers and scientists work closely with the medical community. By engaging with pathologists, clinicians, and other medical experts, the technical team gains valuable insights into the nuances of medical data, diagnostic workflows, and clinical challenges. This interaction ensures that the AI medical device design and implementation are guided by practical, real-world healthcare requirements and meet the most relevant needs of patients and their doctors.
The figure above highlights the conference posters and peer-reviewed articles that were produced along the path to developing MSIntuitⓇ CRC. These publications showcase both the scientific rigor and collaborative efforts involved. Posters provide an opportunity to present early findings and gather feedback from the broader medical and scientific communities. Peer-reviewed articles ensure that experts in the field vet the methods and conclusions. These contributions validate the model’s development and advance the collective understanding of AI’s role in medicine.
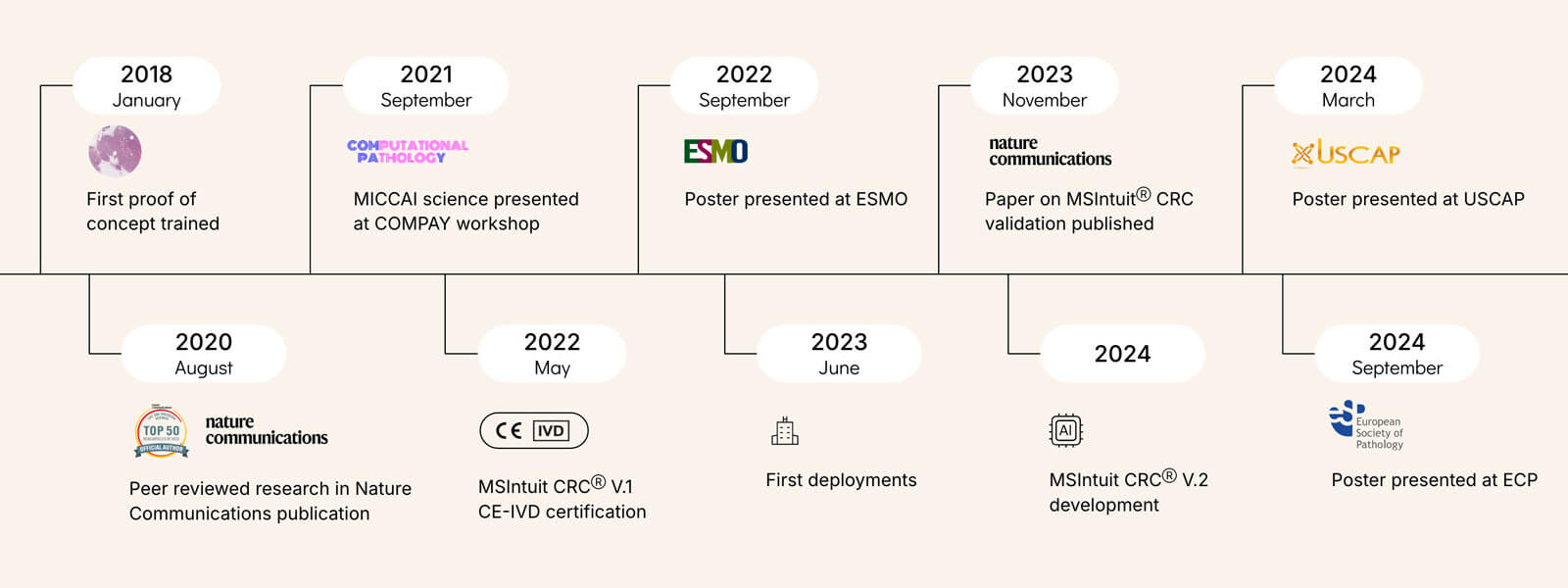
Summary
AI models as medical devices require a thorough validation process to ensure their accuracy, reliability, and safety in real-world settings. Variations in patient populations, lab environments, and equipment, such as pathology slide scanners, can influence a model's performance, making validation of diverse data crucial. The key to a successful AI medical device is ensuring these variations do not degrade the model’s effectiveness. In the next blog, we’ll touch on the regulatory process and distribution of an AI diagnostic.