Blog 1: Opportunities and Obstacles for AI in Pathology
Over the past year, many of us have begun interacting with AI tools regularly with little understanding of how they work. We don’t really need to know how ChatGPT responds to our questions or how our photos app tags our family and friends. And if it gets it wrong sometimes, not a big deal – we might even laugh about it.
But AI is also being integrated into medical applications where the stakes are much higher. To recognize the advantages of AI tools and rely on their results in your everyday work, you need to understand how these tools are developed for pathology. With this understanding, you can make informed decisions about their use.
The evolution of tools for pathology

Hematoxylin and eosin have been the backbone of diagnosis in pathology for more than a century. This staining technique highlights nuclear and cytoplasmic structures, enabling pathologists to easily see the distribution of cells and the tissue’s overall layout. These color patterns are key in creating an interpretable view of tissue structure.
The more recent advent of slide digitization has brought new efficiencies through the use of telepathology for remote consultations and second opinions. It has also helped to democratize education and training in pathology. The adoption of whole slide imaging has been slow due to the upfront costs, but the long-term benefits of improved workflows and collaboration capabilities are increasingly being recognized and are expected to drive broader adoption in the future.
The tools and techniques for digitization have enabled the collection of larger repositories of H&E images. This data is the key to unlocking the next generation of tools for pathologists, tools that can provide an additional boost in interpreting patient samples.
The next generation of tools enhanced with AI
H&E does not always provide enough information to pathologists. Sometimes, they rely on more specific stains, although this can be time-consuming and costly. Techniques like immunohistochemistry and immunofluorescence can highlight different patterns in the tissue compared to H&E and molecular tests can provide insights from RNA or DNA. These methods provide a complementary way to interpret tissue samples.
As the tissue patterns of interest become more complex and the volume of whole slide imagery far greater than any human could review, we next turn to computational techniques using AI to provide a more decipherable view. The specific type of AI relevant here is machine learning. Machine learning uses data to learn patterns and make predictions without being explicitly programmed. It has different strengths and weaknesses than us humans. AI does not have the years of practical experience and weighing different points of view that a pathologist has. But it can process data much faster than humans and find more complex patterns. This makes AI uniquely suited to providing additional mechanisms for interpreting tissue.
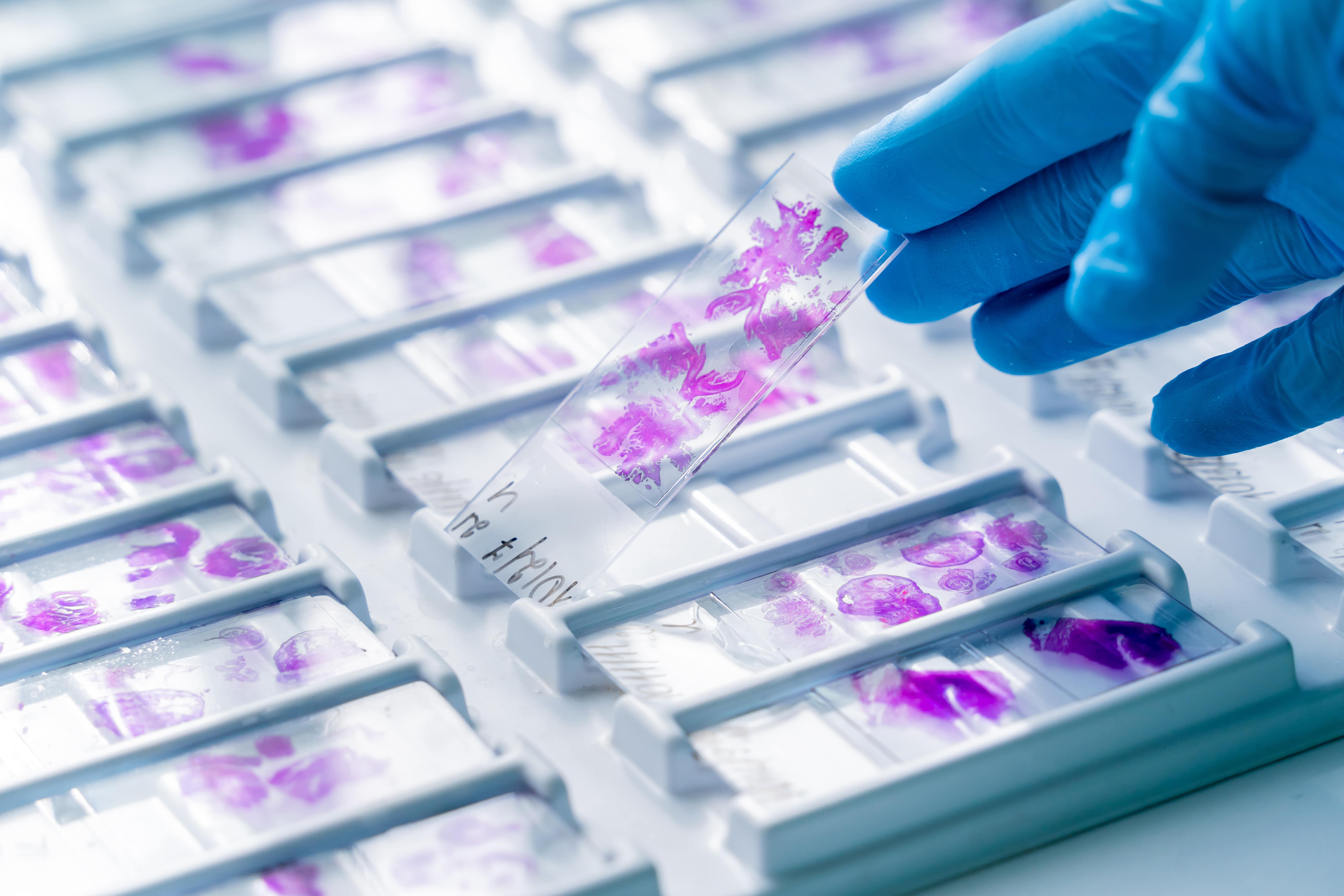
Artificial intelligence allows the exploitation of tissues beyond the interpretation that the human eye can make. In this way, we are breaking new ground and discovering new morphological aspects, thanks to new tools such as artificial intelligence.
– Professor Frederic Bibeau, Pathologist at CHU Besançon, France - Read the full testimonial
AI can augment pathologists in various ways. AI tools can enable more efficient workflows by automating routine tasks like cell counting and measuring tumor size. They can detect subtle patterns and anomalies in tissue samples that might be overlooked by human eyes. This leads to more accurate diagnoses, particularly in complex or rare cases, and helps in identifying diseases at earlier stages, potentially improving patient outcomes. In addition, AI tools can speed up drug development by aiding researchers’ decision-making. Additionally, in the case of companion diagnostics and clinical trials, AI can support the stratification of patient cohorts. Predictive and prognostic tools can also lead to more informed decisions about patient care.
Obstacles to AI tool adoption
The implementation of AI in pathology also comes with some challenges and limitations. One major concern is the initial setup cost and integration with existing laboratory information systems. Furthermore, there are concerns regarding the reliability and accuracy of AI tools, particularly when they are trained on biased or non-representative datasets.
Additionally, the integration of AI into pathology workflows requires careful consideration of ethical and legal issues. Data security and patient privacy are paramount, as the use of AI involves handling sensitive medical information. Ensuring compliance with data protection regulations and safeguarding against security threats are critical challenges.
Despite these challenges, the potential benefits of AI in pathology are substantial. With a rigorous development process and prudent integration, these technologies can significantly enhance the field, driving better diagnostic practices and ultimately improving patient care.
Building trust in AI within the medical community
As the utility of AI in pathology grows, many pathologists are beginning to see AI not as a replacement for their expertise but as a complementary tool that augments their capabilities and improves patient outcomes.

The pathologist will be in front of his screen, he will run his algorithms which will provide him with various probabilities,this will help him, to obtain a more precise and more effective diagnosis. AI diagnostic assistance will also save time because it will be immediate, faster and can be more efficient than our current techniques.
– Professor Jean-Christophe Sabourin, pathologist at CHU Rouen, France - Read the full testimonial
AI systems continue to demonstrate their reliability and effectiveness in clinical settings, and trust in these technologies is growing within the medical community. AI automation allows pathologists to focus on more complex and nuanced aspects of diagnosis and patient care.
Fundamentally, AI is a tool for identifying more complex patterns and providing a more interpretable view of patient samples. In many ways, it serves a similar objective as H&E staining did a century ago, although there is one major difference: the development of these tools is complex and integrates a whole different field of study into pathology.
Owkin is working to deliver AI diagnostics that integrate seamlessly into the digital pathology workflow to support accurate diagnosis at a fraction of the time and cost of existing tests. It’s solutions help improve pathology workflows, pre-screen for biomarkers, and predict outcomes— giving healthcare providers a fuller picture of a patient’s disease. This means more patients can benefit from targeted therapies, making precision medicine more accessible to more patients at an earlier stage of their disease.
What’s next
The advantages and challenges of AI tools are often obfuscated by the complexity of this technology. This blog series will demystify the development of an AI tool for diagnostics. First, we will cover the fundamental role of data, how it is obtained, and the challenges in ensuring quality and security. Next, we’ll lay out how models are built and the different ways to learn from data. Following this will be product validation, where we’ll discuss the different types of validation and their essential roles. Then comes a review of strategies for market access and distribution to pathologists. Finally, we’ll talk about integrating the AI device into the pathologist workflow.
Stay tuned for the next blog of the series