Getting it right: a mindful approach to healthcare AI
Technologies and analytical techniques falling under the umbrella heading of ‘artificial intelligence’ clearly have enormous potential to significantly improve the quality, efficiency, and effectiveness of healthcare services, and so, ultimately patients’ health. When harnessed correctly, AI can provide support to human clinicians, considerably enhancing their diagnostic, prognostic, and predictive capabilities. This can help to make medicine more proactive and personalized, presenting healthcare systems across the world with an opportunity to potentially save lives. This opportunity should not be ignored. Although AI itself is not new, its use in the delivery of ‘frontline’ care is very much in its infancy and, as such, it is a very new ‘type’ of technology—one that healthcare providers have not really had to contend with before.
AI is far more complex than, for example, a new form of blood test; it is a system-level technology. In other words, the use of AI changes the entire healthcare pathway because it fundamentally disrupts the Hippocratic Triangle.
Set out by the father of Western medicine—the Greek physician Hippocrates—the Hippocratic Triangle refers to the relationship between three key elements of healthcare: the disease, the patient, and the physician. Safeguarding this relationship still underpins the Hippocratic Oath today.
Whereas once ‘diagnosis’ was a process that primarily involved one patient and one clinician in one location, when AI is added to the mix, it becomes a process involving thousands of patients and their data. It also involves multiple mediating parties: those collecting data, curating it, using it to train AI models, and so on. It also involves an ‘algorithm’ and either clinical decision support software or some form of customer-facing app. Many of these newly involved parties exist outside the healthcare domain, and algorithms themselves are incapable of embodying the uniquely human traits most important to healthcare, such as empathy.
This disruption to the Hippocratic triangle means that, as much as AI presents enormous opportunities for healthcare, it also presents risks to its long-held ethics—to the well-known concept of ‘do no harm.’ These risks must be proactively managed by encouraging all those involved in developing, deploying, and using AI for healthcare to be ‘ethically mindful.’ The first step in developing this particular attitude is to identify the ethical risks proactively and clearly. It is this task that I intend to help with here.
Making reasonable decisions with AI
Any AI tool used in healthcare, whether it is being used for image recognition, prediction, or clinical decision support (i.e., helping a doctor to diagnose a patient and identify the most suitable treatment), is trained on data, including demographic and socioeconomic data; symptom and existing diagnosis data; treatment data; outcome data; and other ‘omic’ data (such as genomic data). Much of this data was not collected for research purposes, it was collected for the purpose of helping clinicians care for patients in clinical settings.

It is, therefore, far from perfect. Health data might, for example, be missing key information such as a patient’s ethnicity, or it might be incorrect. Most electronic health records (EHR), for example, rely on ‘coded data.’
When a doctor diagnoses a patient they use a ‘code’ (typically a series of numbers) to record that diagnosis. For example, in England a diagnosis of ‘Urinary tract infectious disease’ (commonly known as a UTI) is recorded as 1090711000000102.
Sometimes, because clinicians are busy and EHRs are not always easy to use, the wrong code might be used. Other errors might be even simpler, for instance, a person’s height or weight might be incorrect which, in turn, might mean that theirBMI (body mass index) is improperly calculated.These types of problems are not always easy to catch—especially when the data is being used by those outside the clinical community. Unless corrected through careful data curation these types of errors can mean that AI tools learn the ‘wrong thing,’or provide the ‘wrong advice.’
It is perhaps best to illustrate these risks with a very simple example from the Covid-19 pandemic.In England, research into the ‘factors associated’ with Covid hospitalization or death showed that individuals with a higher BMI were at greater risk of severe outcomes than individuals with a lower BMI, among other factors. When it came to rolling out the Covid vaccine then, individuals with higher BMI were considered higher risk and were, consequently, higher up the prioritization list—policy makers decided that they should be offered the Covid vaccine earlier than others. GPs were provided with a very basic ‘algorithm’ they could use to identify all their patients who should be invited to be vaccinated early in the roll-out process. In one instance this algorithm identified a man called Liam Thorpe as being high risk for Covid and so he was invited to be vaccinated in early February 2021. Thorpe was surprised to receive the invitation as he was, in fact, a healthy man in his 30s with no underlying health conditions and a ‘normal’ (i.e. not elevated) BMI. It was only when he queried the invitation that it was discovered that he had been recorded as being 6.2cm tall in hisEHR, and thus the algorithm believed he had a BMI of 28,000 (‘healthy’ BMI is between 18.5 and 24.9).Although this example does not really involve any AI, it illustrates how a model trained on, or presented with, inaccurate data might make poor decisions—in this case ‘over-diagnosis.’ It could equally have illustrated the alternative of missed diagnosis ifThorpe had, in reality, had a very high BMI but his height or weight was not recorded in his EHR, so he was missed entirely by the ‘prioritization algorithm.’
In other instances, AI tools might be trained on data that is far too specific—this is sometimes known as ‘overfitting.’ A very famous example of this exists outside healthcare, where an image-recognition algorithm was being trained to differentiate between wolves and huskies. During training, the algorithm was performing well, but when it was shown images that it was not shown in training it started to perform quite poorly—missing obvious examples of wolves and misclassifying them. When the researchers tried to work out why this was, they realized that what the algorithm had learned to recognize was snow. During training every wolf image that the algorithm was shown had snow in the back-ground. So, the algorithm had learned that snow = wolf, and when it was shown an image of the same animal with no snow in the background, it didn’t recognize it as a wolf. The same type of problem could (and has)occurred in healthcare. For example, if an algorithm learning to identify cancerous breast cancer tumors is only ever shown cancer-containing CT-scans taken on a particular CT-scanner it might learn to associate cancer with the type of CT-scanner, rather than the type of tumor, and so struggle to recognize cancer when it is shown a scan taken using a different type of scanner.
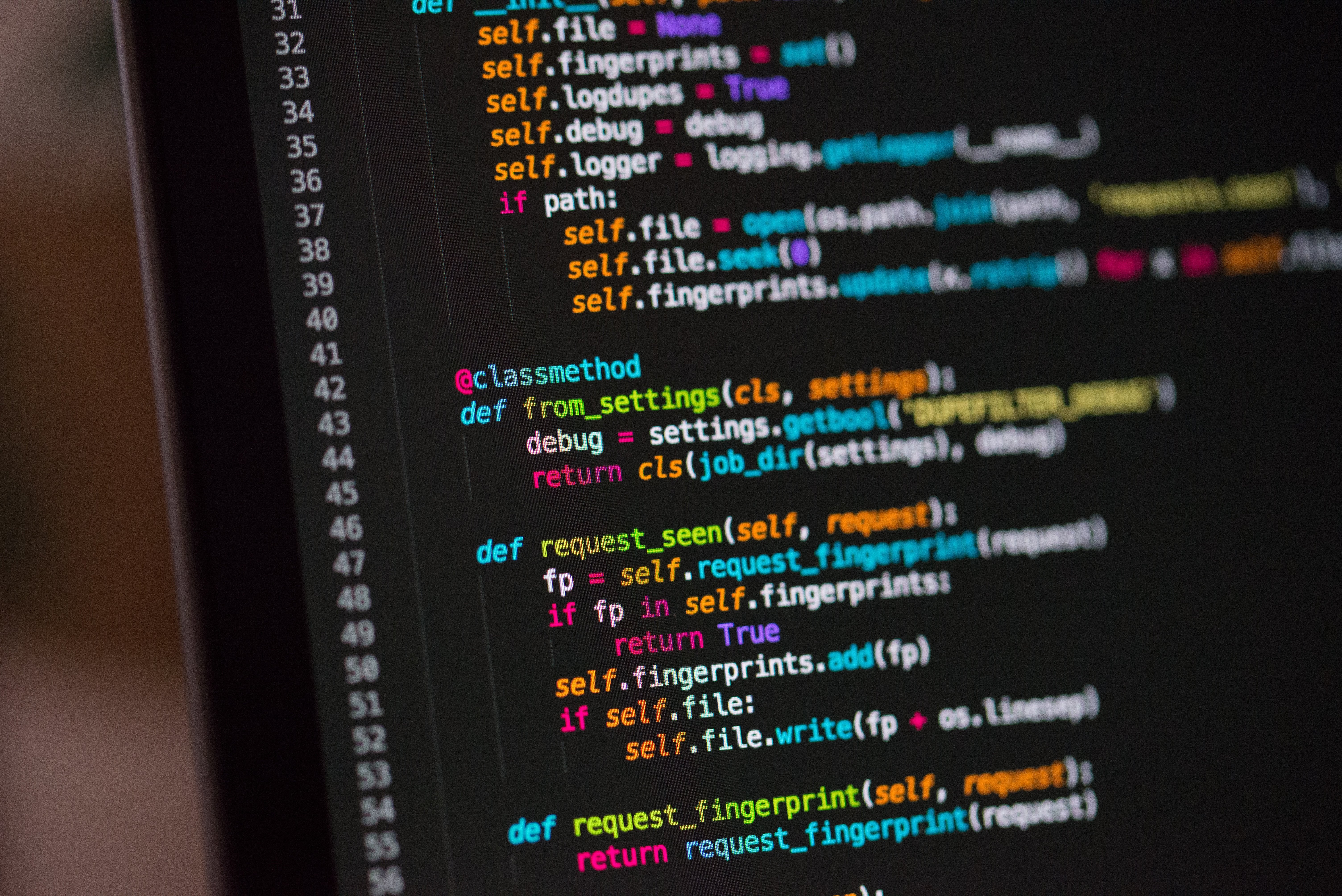
A final data-related problem comes from the fact algorithms do not understand context—or to put it another way, just because an algorithm can identify a pattern it does not necessarily mean that pattern is meaningful or helpful. A well-known example of this comes from the 1990s when researchers in Pittsburgh set out to develop an algorithm that could predict whether a patient admitted to hospital for pneumonia would develop serious complications.
Artificial Neural Networks structure multiple operations in layers, facilitating a form of machine learning called deep learning. Inspired by processes undertaken by the human brain (although, they work very differently in practice) these networks are capable of incredibly sophisticated analysis and predictive tasks. For more on this process, see our feature on ResNet.
The algorithm—in this instance a neural net—started to produce very curious results. It was suggesting that pneumonia patients who also had asthma were at lower risk of complications. This is, of course, incorrect; patients who have asthma are at much higher risk of complications from pneumonia. The problem was that, in the past, asthmatic patients who were admitted with pneumonia were triaged and given more medical attention early on than non-asthmatic patients. As a result, they had better outcomes—because they received better care. But the algorithm didn’t understand this context, so had incorrectly interpreted the historical data it was trained on as suggesting people with asthma had lower risk of developing pneumonia-related complications.
These are all examples of ‘epistemic’ ethical concerns related to AI, or ethical risks associated with the ‘knowledge’ used by algorithms to make decisions. When the knowledge isn’t carefully considered, significant ethical risks of missed diagnosis, over-diagnosis, mis-diagnosis, and more, can result—all of which can cause considerable harm to individual patients.
Ensuring AI is fair
The above examples give the impression that every-one is at equal risk of having an algorithm mis-,under- or over-diagnose them if it is poorly trained, and, therefore, everyone is at equal risk of ethical harms resulting from algorithmic decision-making in clinical care. To a certain extent this is true.
The Liam Thorpe example shows that anyone who has an error in their EHR is potentially ‘at risk.’However, the unfortunate truth is that certain groups of individuals are at higher risk of ethical harms than others.
Again, to a very large extent, the problem here lies in the data used to train—or to develop—AI systems used in healthcare. Traditionally, healthcare has collected more data on, for example, individuals who are white, cisgendered, heterosexual, and middle class. These individuals have an easier time accessing healthcare and, often—awful as it is—have better quality outcomes from healthcare. The health data reflects this. Unless this ‘bias’ is carefully managed, algorithms trained on health data are, therefore, likely to be more accurate for individuals who are white (and cisgendered, etc) than those who are not. People of color, people who fall outside the gender binary, and people from the LGBTQ+ community are, therefore, at higher risk, of missed diagnosis, under-diagnosis, over-diagnosis, mis-diagnosis, or any other instance of poor-quality algorithmic decision-making.
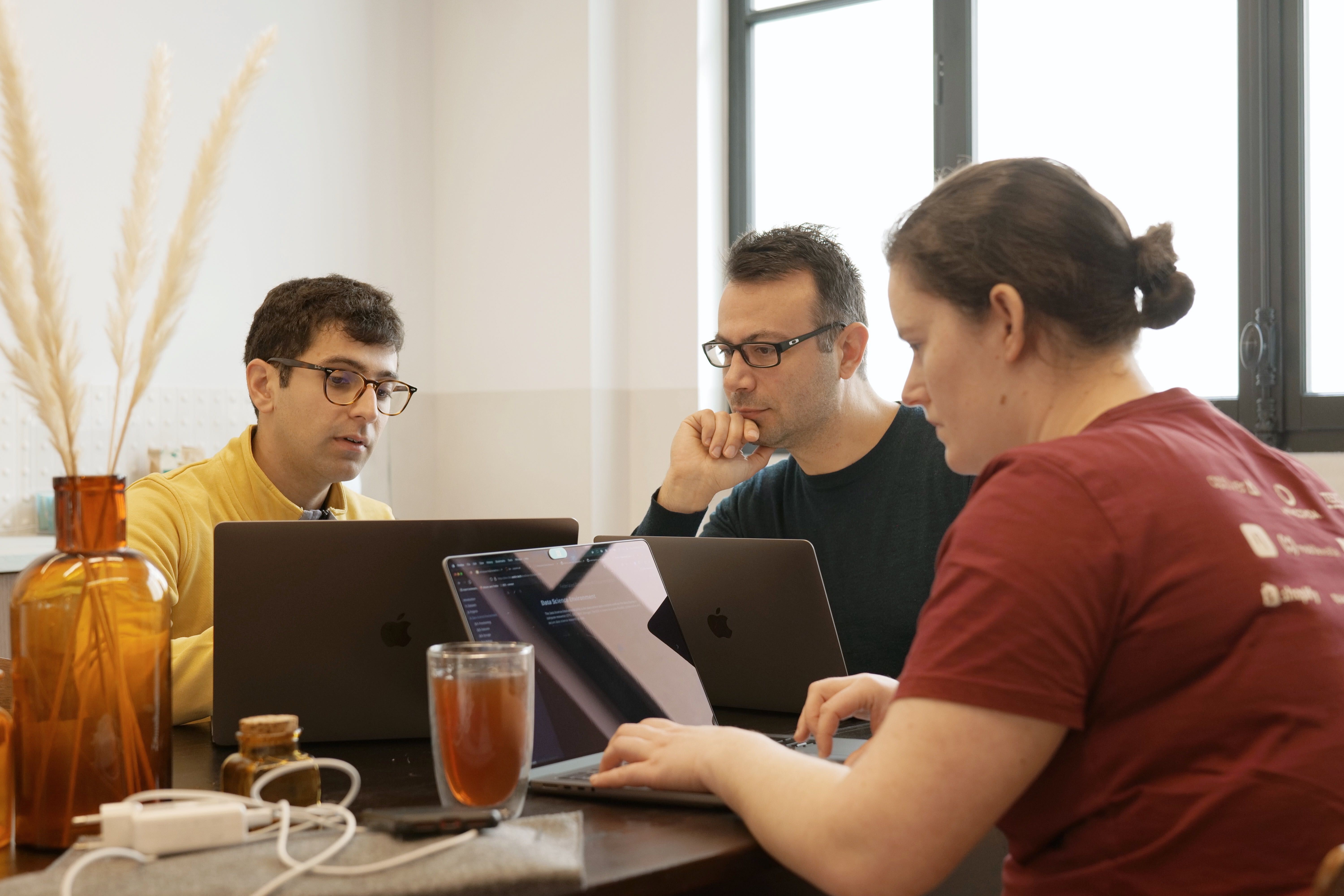
The risks of such biases have already been illustrated outside healthcare, most notably in the criminal justice system. A high-profile publication by ProPublica, for example, demonstrated that black individuals were more likely to be rated—by a decision-making algorithm—as being at higher risk of ‘recidivism’ (or re-offending) than their white counterparts, even when other circumstances(including the severity of the original crime) were the same. The result of this was that black individuals were more likely to be given harsher sentences than their white counterparts. The underlying issue? Well, historically judges have been biased themselves and often treated black individuals unfairly—for example, handing down harsher sentences for seemingly petty crimes. The historical data that the decision-making algorithm was trained on therefore ‘taught’ it that black individuals were higher risk.
If we are not vigilant, a similar scenario could arise in healthcare. Consider, for a moment, an algorithm that is being developed to predict the likelihood of an individual developing prostate cancer. The dataset that it was trained on contains mostly data collected from white cisgendered men.Non-white and non-cis individuals with prostates are not well represented. The algorithm learns, with a very high degree of ‘sensitivity and specificity’ the risk factors associated with developing prostate cancer for white cis males, and so the researchers responsible for developing the algorithm believe that it is performing well and so deploy it in clinical systems across the country. Healthcare providers use the algorithm to ‘screen’ individuals who might be at higher risk and start offering the identified individuals proactive and preventive care. What the healthcare providers do not realize is that the algorithm is far worse at predicting the development of prostate cancer in individuals who do not fit the white cis-gendered profile.These individuals are far less likely, therefore, to be identified in the algorithmic-screening process and, consequently, far less likely to be offered proactive interventions—that is, the quality of care for men of color is far lower and so their outcomes are far poorer.
Related to this is the issue of ‘blame.’ In healthcare, as in the preceding example, one of the main uses of AI is prediction. The idea being that if risks of health deterioration can be predicted earlier, then individuals might be able to take action to lower their risk. The subtle implication of this is that if you are given the opportunity to lower your risk of developing diabetes, for example, and yet you do not act, take insufficient action, or the actions you do take are ineffective, and—as a result—you develop diabetes, then it is somehow ‘your fault’ for failing to manage your health appropriately.
There are multiple problems with this logic, but the main one is that the ability of an individual to take action to lower their health risk profile is almost entirely dependent on their wider circumstances—their so-called ‘social determinants of health.’
The social determinants of health (SDH) encompass a range of non-medical factors that can significantly impact medical outcomes.It’s only in recent years that SDH—which encompass and refer to the complex conditions in which people are born, grow, live, work, and age—have started to be properly understood.
Risk factors forType 2 Diabetes, for example, include being overweight and/or being physically activeless than three times a week. Individuals with ‘prediabetes’, or other-
wise identified as being at higher risk of Type 2 Diabetes, might, therefore, be encouraged to lose weight and become more physically active. This may seem simple enough except for the fact that multiple socioeconomic factors impact upon an individual’s ability to lose weight. Fast food is, for example, far cheaper than fresh lower-calorie food; individuals who work multiple jobs or work in shifts might find it difficult to find time to exercise.Likewise, individuals who are responsible for caring for others—whether that be children or other dependents—might also find it harder to exercise or prepare lower-calorie, more nutrient-dense food. Individuals on lower incomes, with multiple care dependents, working multiple jobs, might, therefore, find it significantly harder to reduce their risk of Type 2 Diabetes than individuals with other circumstances. Should these individuals then be ‘blamed’ if they do go onto Develop Type 2Diabetes? Of course not, but we cannot deny the risk of that happening—especially as healthcare systems across the globe face increasing resource restrictions and may be forced to make ethically difficult decisions. Thus, we can see that just as there are ethical risks associated with the knowledge thatAI systems are trained on, there are ethical risks associated with the impacts—particularly potentially unfair ones—of AI systems used in healthcare.
Holding AI to account
The final group of ethical risks associated with the use of AI in healthcare relate to ‘accountability.’ In medicine, where mistakes can mean the difference between life and death, it is very important that when something goes wrong the reason for the error is identified. Identifying the source of error reduces the risk of it being repeated and harm coming to multiple individuals, or multiple groups.
If, for example, a patient has an adverse reaction to a drug prescribed to them by a doctor, it is crucial to know: whether the patient was allergic to the drug and this was previously unknown; if the reaction was due to a dosing error; if the reaction occurred only because the patient was taking another drug at the same time; or if the drug routinely causes adverse reactions in certain groups of people.Healthcare providers and regulators have, therefore, devised multiple mechanisms for mitigating the likelihood of errors occurring, identifying errors in as close to real-time as possible, and for dealing with the aftermath of an error.
Some of these mechanisms are legal or regulatory (such as medical device law), some are technical (such as the Yellow Card scheme for report-ing adverse reactions), some are sociocultural (such as the benchmarking aspect of medical negligence cases, where doctors of the same grade as the doctor ‘under inspection’ are asked if they would have made the same decision in the same context). The exact mechanisms are, however, irrelevant; what matters is that there are well-established means of identifying potentially harm-inducing errors and holding the responsible individuals, organization or product to account. This ‘safety-net’ does not yet exist (at least not in a reliably tried-and-tested way) for health tools falling under the umbrella heading of AI.
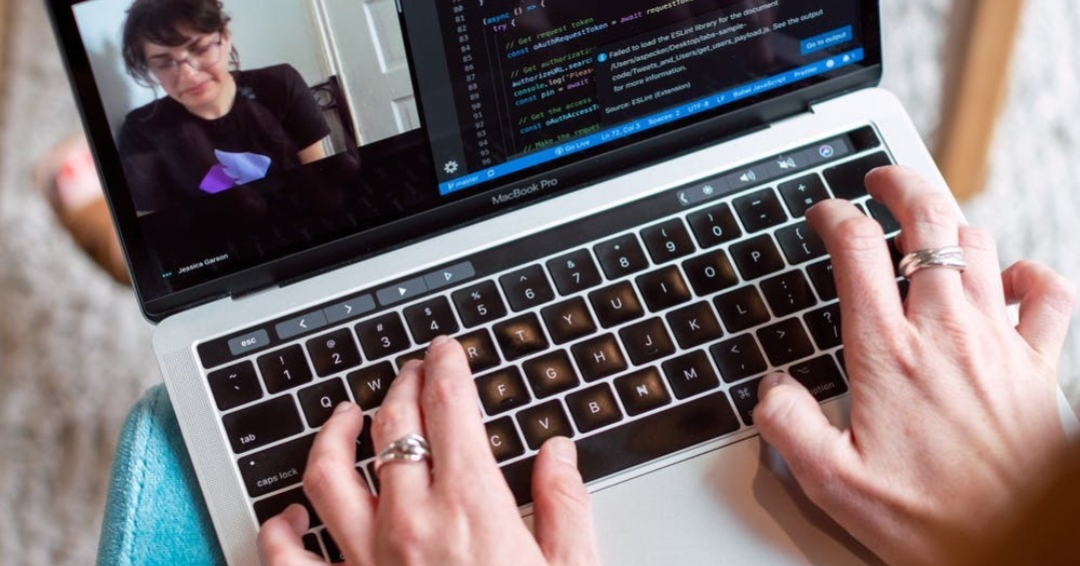
Consider again the algorithm being used to predict prostate cancer risk so that individuals who are identified as high-risk can be offered proactive care. Imagine that the algorithm has miscalculated the risk of one individual as being low. Because of this ‘low-risk rating’ the person in question is not offered proactive care (such as screening) and they feel sufficiently reassured that they themselves do not need to pay close attention to the potential symptoms of prostate cancer. Imagine that this individual then goes onto develop prostate cancer but, because neither they nor their doctors were paying particularly close attention, this goes undiagnosed until stage 4and the likelihood of the cancer being ‘curable’ is significantly less.
It would be very difficult in this scenario to identify the source of the error. Was it in the training data (as discussed above)? In which case, should those responsible for collecting, curating, and providing the training dataset be held accountable? Was it in the patient’s EHR, meaning the risk-screening algorithm reached an erroneous conclusion (as in the Covid vaccination example)? In which case, should the designer of the EHR be held accountable, or perhaps the person responsible for miscoding a vital piece of information in the record? Was it in the doctor’s interpretation of the results of the risk-scoring algorithm? In which case, should the doctor be held accountable? Or perhaps it was in the ‘productization’ of the risk-scoring algorithm, and the way in which it was implemented into the clinical system, in which case maybe it’s the manufacturing company that should be held accountable?Alternatively, maybe the fault was further up the chain and the model selected for the risk-scoring algorithm was an inappropriate choice for the task at hand, and so the data scientists should be held accountable? The list could go on. The point is to illustrate the fact that product pipelines for AI ares o complicated that errors are often untraceable.Consequently, they are difficult to detect and rectify—especially when it is very unclear how the existing legal system would cope with a case of ‘algorithmic harm.’ (Although it should be said that such ethical complexities have always existed in healthcare, as in any complex system where decision-making is diffuse).
The ethical risks associated with this lack of traceability are significant and manifold, but primarily they relate to scale and trust. To tackle scale first, although there have been cases in theUK and further afield of one doctor, or one hospital, causing harm to multiple individuals, these cases have been few and far between and are very much the exception, not the norm. With the introduction of AI tools, the risk of one ‘rogue algorithm’ causing harm to multiple—maybe even thousands—of individuals before the fault is detected, corrected, and the harm rectified, is much higher. This risk is compounded by the fact that some early evidence suggests that clinicians might, themselves, feel unable to question the results of AI tools, even if the results seem clinically spurious, because of the effects of ‘automation bias.’
The tendency for humans to perceive the outputs of any automated decision process (in this case, an AI tool) as being more consistently reliable—and therefore potentially more accurate—than outputs of human decision-making processes.
Thus, we may find ourselves in a situation where an error in an AI tool goes undetected for a long period of time, and is then difficult to trace, correct, and rectify—and thus, difficult to prevent from reoccurring. In the meantime, many thousands of patients may have come to harm as a result.
This brings us to the final, and perhaps primary issue: trust. Healthcare is a system that is entirely reliant upon trust. Patients need to be able to trust that their doctors have the skills, training, and knowledge to treat them appropriately, and that they have their best interests at heart. Doctors need to be able to trust the tools that they are using to help them diagnose and treat their patients. Both patients and doctors (and of course others involved in healthcare) need to be able to trust that if things go wrong, they will be caught by a sufficiently robust safety net. As soon as any one of these trust links is broken, the potential consequences for individual patients, doctors, specific groups, organizations, the healthcare sector, and possibly even society, are severe.
It is no real wonder then that current low trust in AI tools among patients and doctors is acting asa major barrier to their adoption and implementation, and why many well-designed, potentially safe and efficacious AI tools are residing in the ‘model grave-yard,’ rather than helping to improve frontline care.Fortunately, there are steps that can be taken to mitigate the outlined ethical risks and build trust.
Asking the hard questions
Hopefully it is clear from this brief overview that as much as AI presents enormous opportunities for ‘doing good’ in the healthcare system, it also presents some significant challenges to those who try to govern healthcare—those who are responsible for developing the legislation, regulation, policies, and ethics frameworks that surround healthcare. Design decisions about how AI tools are developed, deployed, and used have wide-ranging implications, from the efficacy of the healthcare systems to the values underpinning society. To mitigate these risks and ensure that healthcare systems can capitalize on the myriad opportunities presented by AI for health—in a way that is socially acceptable and ethically justifiable—regulators, legislators, policy makers, and others will have to take steps that go beyond data protection or medical device law. Such ‘hard’ mechanisms only dictate what can or cannot be done within the bounds of the law; they do not help guide decisions about what should or should not be done. This is where being ethically mindful comes in.
All those involved in the AI-for-health-care pipeline need to be provided with framework mechanisms that help them think through the big ‘should’ questions.
Such overarching frameworks could include: how much clinical decision-making should we be delegating to AI solutions? Which values should be embedded in algorithms?
Once these frameworks are in place, attention should turn to pragmatic questions such as: how can we ensure doctors still maintain an appropriate degree of autonomy once AI is embedded? How are key tenets of ethical healthcare (such as empathy) protected? How can we ensure healthcare is still used as a means of promoting social justice? How can we ensure the values and priorities of individual patients are still accounted for in different decision-making? How do we ensure we do not overfit the healthcare system, so it suits only one ‘type’ of patient? And so on.
These questions should not be asked and answered in isolation, but with the active involvement and inclusion of all individuals, groups, and professions potentially impacted by the use of AI in healthcare. These individuals and groups must be seen as key ‘designers’ of AI tools and the system as a whole. Their view must be seen as being as important as well-curated datasets: they must not be seen asa problem to overcome or another box to tick.
If all those involved in the development, deployment, and use of AI tools for healthcare can be encouraged to take these steps, to actively involve all impacted parties in decisions all the way from ideation to implementation and beyond; if, as a system, we can pause long enough to ask the difficult questions and ensure we’re being mindful of the potential ramifications of introducing a new entity into the ‘Hippocratic Triangle,’ then the future is bright. Then, and only then, can the true benefits of AI for healthcare be realized.
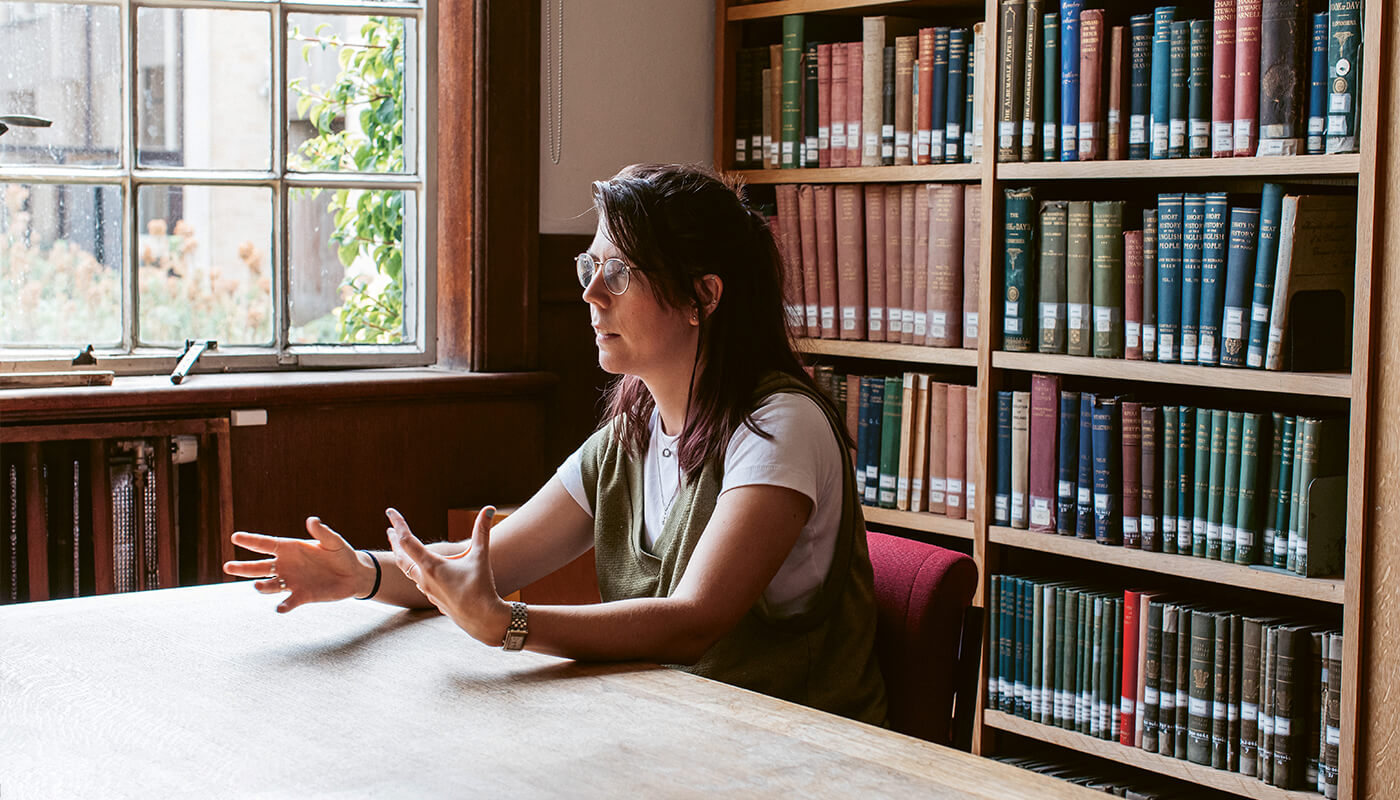