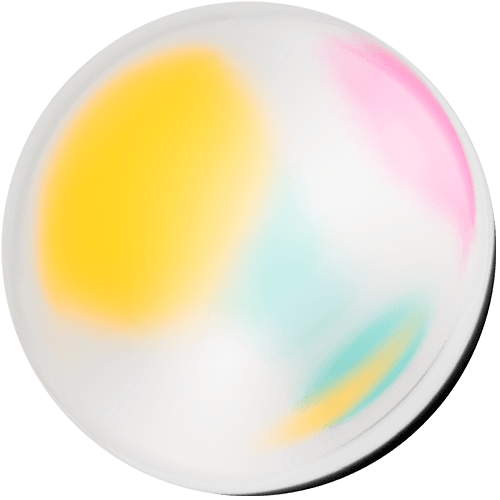
Scientific publications
Our latest science, research and ideas
We publish our cutting-edge AI research in peer-reviewed journals and at world leading conferences
Explore
Thank you! Your submission has been received!
Oops! Something went wrong while submitting the form.