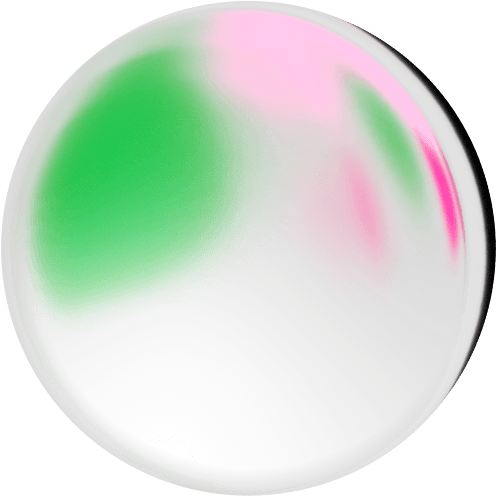
Data
Join our Federated Research Network
Our growing patient data and KOL network
with 50% of the world's top 20 medical institutions
Clinical data, single-cell and spatial omics, and histology
Working with top academic centers to access AI-ready patient data
Unparalleled depth of data from hard-to-find cohorts
Our approach to data
Owkin works with top academic centers to curate, enrich, and generate deep, multimodal data that is AI-ready
Challenges
Barriers to develop and validate AI across datasets
Owkin’s solutions
Data services for Owkin sponsored projects
Owkin helps academic partners to upgrade their data to accelerate their AI research in real world healthcare settings. Our data services:
Accelerating research
We combine privacy-enhancing technologies, like secure data aggregation and federated learning, with critical clinical expertise and a network of academic researchers.
Federated learning software breaks medical data silos and accelerates innovation in real-world healthcare settings.
Federated learning
Connect disjointed data to build accurate models without pooling data
Spotlight
Early career researcher
Owkin collaborates with Charlotte Spencer, CRUK Clinical Research Fellow at the Francis Crick Institute, to apply the latest machine learning techniques to discover kidney cancer biomarkers.
Are you an early career researcher interested in incorporating cutting-edge AI in your research?
Healthcare events
Meet our data access experts
Our AI diagnostic solutions and how they integrate seamlessly into digital pathology workflows to support pathologists with accurate and timely diagnosis.
