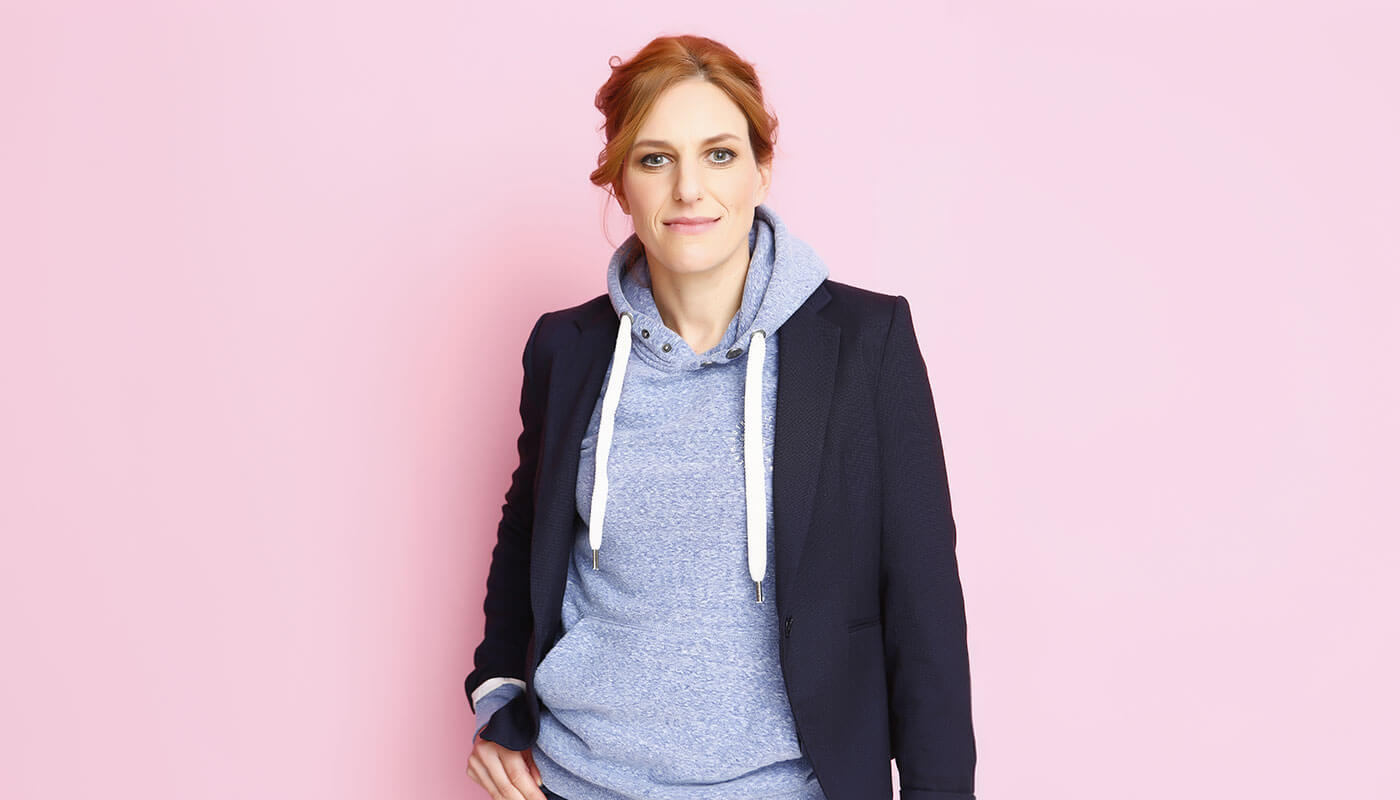
Katharina Von Loga
I have been working with AI for seven years now, and the process of building AI models from whole slide images (WSIs) is both fascinating and complex. At Owkin, we are dedicated to improving the accuracy and efficiency of pathology diagnoses through AI.
The first step in building a model is to convert image tiles into numerical features that capture important tissue characteristics. As a pathologist, I help identify and label key features in the tissue samples, ensuring the model focuses on relevant characteristics. The machine learning model then identifies these features by comparing image tiles and their transformations. This unsupervised learning approach allows the model to compute a vector of features for each image tile.
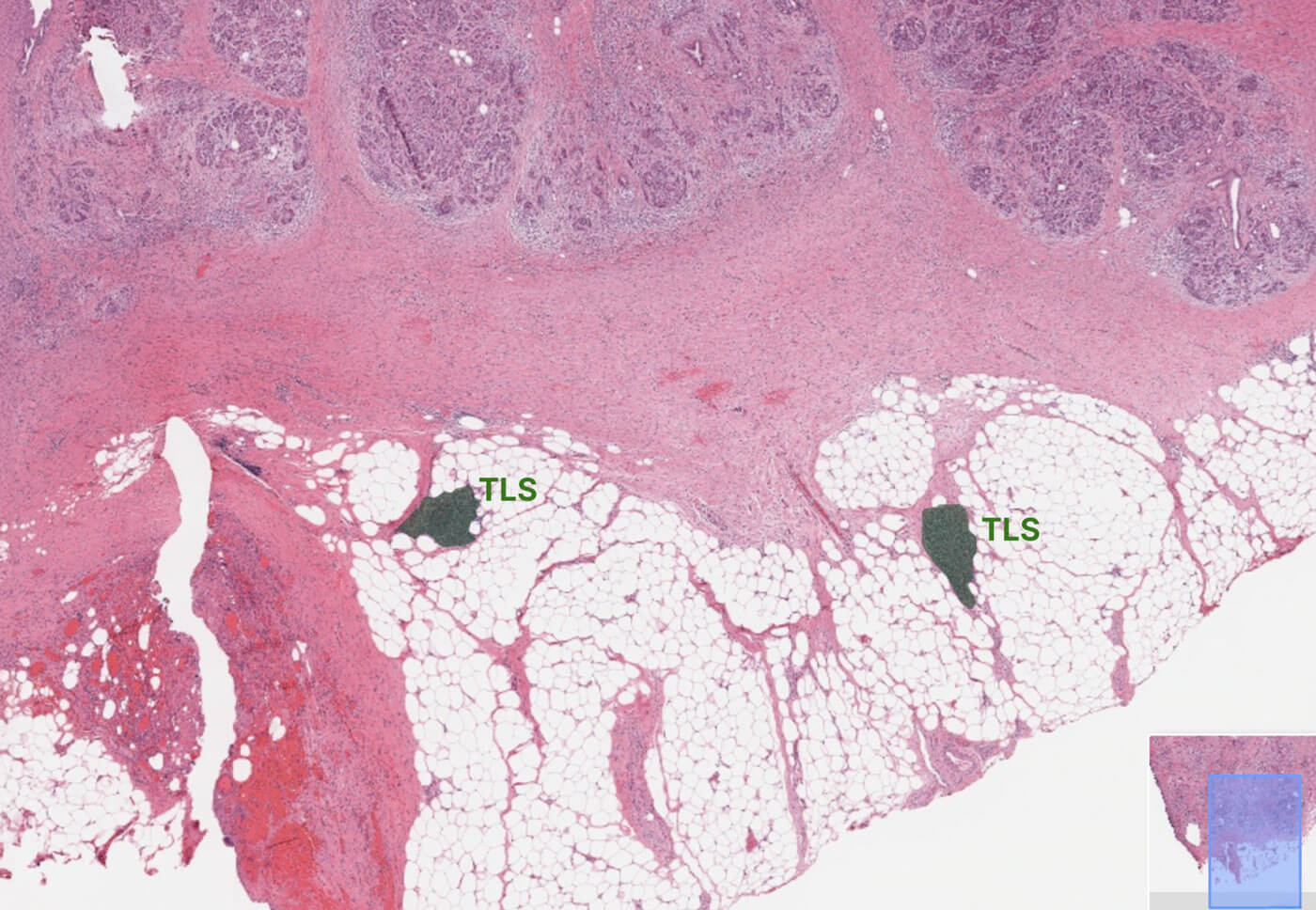

Once trained, these features are used to process slides and make predictions. This involves supervised learning, where the model learns to distinguish between different classes based on the tile features. My role here is to provide expert feedback on the model's predictions, helping to refine its accuracy.
Model interpretability is crucial. For WSI models, interpretability involves highlighting which tiles contributed to a prediction and revealing common characteristics associated with each class. This is often accomplished with a heatmap that highlights tiles associated with each class. By reviewing these tiles, I can confirm known features and discover new ones, ensuring the model's outputs are clinically meaningful.

Developing a machine learning model is an iterative process, requiring many tweaks and experiments to improve performance. The next step is to validate that the model is ready for use in a diagnostic tool, ensuring it predicts correctly and is interpretable.
Our collaboration at Owkin has been instrumental in this process. We work closely with engineers to refine the models, ensuring they are both accurate and interpretable. This collaborative approach allows us to advance the field of pathology through AI, and I am excited to see the continued progress we make together.