Nature Medicine publishes breakthrough Owkin research on the first ever use of federated learning to train deep learning models on multiple hospitals’ histopathology data
The discovery paves the way for AI-powered medical research using larger multi-centric datasets, allowing models to escape the biases of single-centric studies. This has the potential to unlock breakthroughs in precision medicine through the use of secure and privacy-preserving AI.
Using data kept within four leading French hospitals, Owkin built AI models that can accurately predict the future response of triple-negative breast cancer (TNBC) patients to neoadjuvant chemotherapy. By using interpretable AI to extract information from digital pathology slides, Owkin was able to find potential novel biomarkers. This could in the future help funnel patients towards either less toxic treatments or new experimental treatments, improving the personalization of medical care.
The project used federated learning – a collaborative AI framework that preserves data privacy and security through Substra, Owkin’s recently-open sourced software that makes every operation traceable using hyperledger technology. The research is the first time that machine learning models have been trained using histopathology data from multiple hospitals without the data leaving the hospitals. Previously, most studies were restricted to simulating FL by artificially splitting data. It is a landmark proofpoint of FL in medical research and represents a breakthrough in the realization of AI’s practical benefit to research.
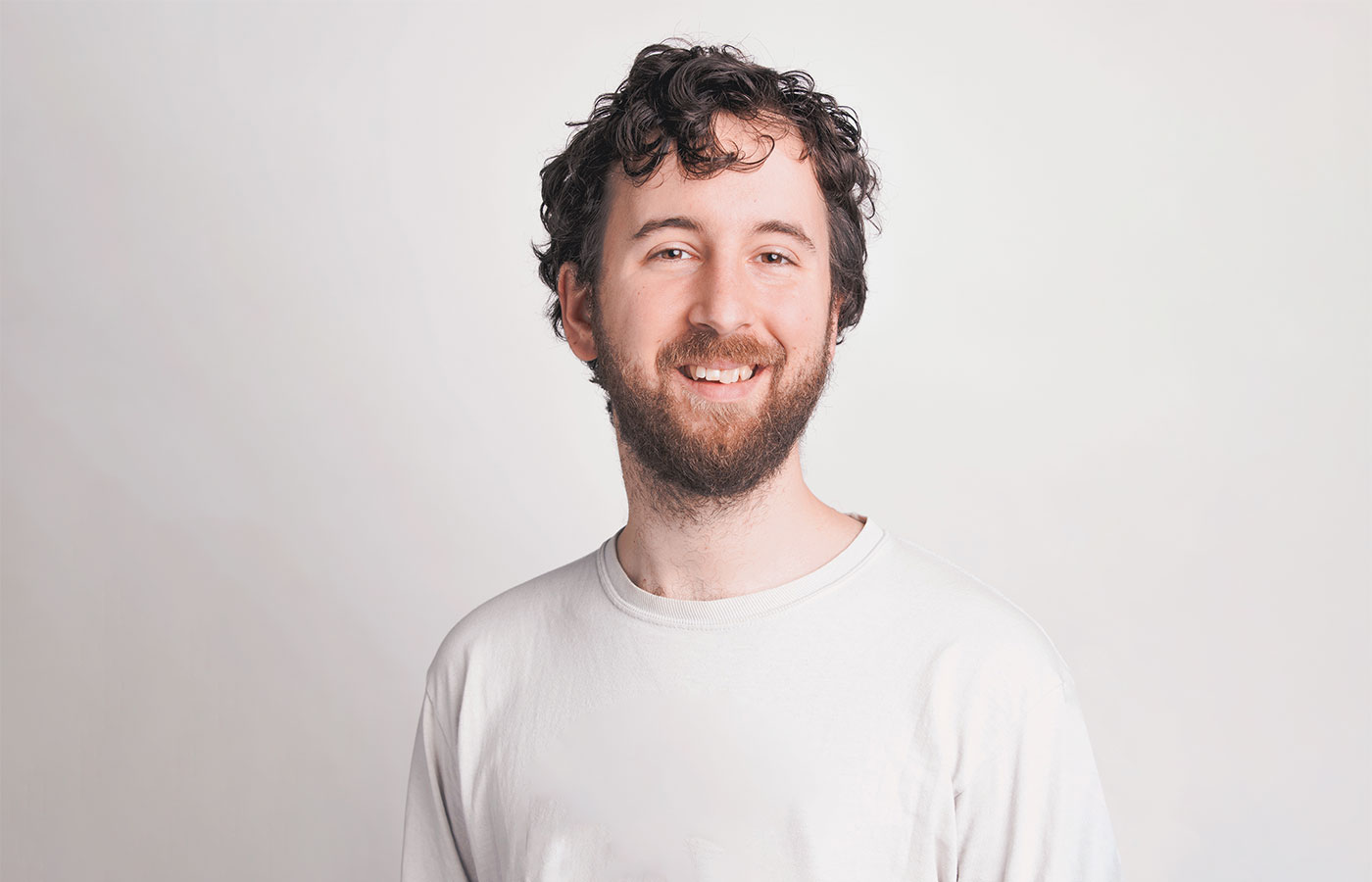
The study used digital pathology data and clinical information from 650 patients from Institut Curie in Paris, Centre Léon Bérard in Lyon, Gustave Roussy in Villejuif and IUCT Oncopole in Toulouse, making it one of the largest TNBC cohorts of its kind ever assembled for such kind of analysis.
The research builds on Owkin’s pioneering use of FL to enable pharmaceutical companies to collaborate on drug discovery research while safeguarding privacy, security and competitive considerations. The MELLODDY project’s results, published this year, showed that collaborating in AI for drug discovery is possible at industrial scale thanks to FL, a first for the industry. In addition to addressing privacy and security concerns, FL can also simplify data governance issues, removing the need to transfer data and fostering more collaborative research.
Jean du Terrail, lead author and Senior Machine Learning Scientist at Owkin, said:
Thanks to our partners, we are proud to have performed an original federated analysis on medical data in real-life conditions, and the first of its kind on histopathology data. By connecting institutions in a federated manner we were able to reach the critical mass of triple negative breast cancer data necessary for the AI to discover, on its own, histological patterns predictive of the response to treatment.
We hope that this proof of concept will inspire medical institutions to collaborate in federated learning networks in order to move research forward while keeping patient data private.
Julien Guérin, Chief Data Officer at Institut Curie in Paris, France, said:
We reached an important milestone with the deployment of this federated learning infrastructure, showing a new cutting-edge approach of building AI in cancer research. We are really happy to have been part of this adventure and hope this will open promising perspectives for the future of patient care.
Dr Guillaume Bataillon, pathologist at IUCT Oncopole in Toulouse, France, and former pathologist at Institut Curie in Paris, France, said:
Through this multidisciplinary partnership, we verify the feasibility of an inter hospital collaborative federated learning approach on a relevant biological question. This allowed us to create pooled heterogeneous dataset in a secure and faster way in order to develop reproductible, transferable and even interpretable models. This proof of concept has the potential to become a tool for therapeutic decision.
Dr Pierre Etienne Heudel, Medical oncologist at Centre Léon Bérard in Lyon, France, said:
The rise of digital pathology coupled with the explosion of different machine learning techniques should enable increasingly precise and personalized medicine. Moreover, the federated learning, achieved in this project by avoiding external data streams, facilitates and secures the process for future daily clinical practice.
Dr Magali Lacroix-Triki, pathologist at Gustave Roussy in Villejuif, France, said:
Digital pathology and AI represent the third revolution in the world of pathology, and pathologists are excited to lead this new change in their practice. Federated learning, pioneering AI research in digital pathology, brings us one step closer to identifying new biomarkers in oncology while ensuring data privacy and security.
Dr Camille Franchet, pathologist at IUCT Oncopole in Toulouse, France, said:
By enabling AI models to be trained on multicentric data without centralization, federated learning unlocks one of the major obstacles in machine learning on medical data with no compromise regarding the respect of personal data.
Authors
About Owkin
Owkin is the first full-stack TechBio company on a mission to understand complex biology and derive new multimodal biomarkers through AI.
We identify precision therapeutics, de-risk and accelerate clinical trials and develop diagnostics using AI trained on world-class patient data through privacy-enhancing technologies. We merge wet lab experiments with advanced AI techniques to create a powerful feedback loop for accelerated discovery and innovation in oncology, cardiovascular, immunity and inflammation.
Owkin also founded MOSAIC, the world’s largest spatial multi-omics atlas for cancer research across seven cancer indications.
Owkin has raised over $300 million through investments from leading biopharma companies, including Sanofi and BMS, and venture funds like Fidelity, GV and Bpifrance, among others.